The power of big data: Smart maintenance using data analytics
Posted: 4 January 2021 | Priya Agrawal | No comments yet
Priya Agrawal, Deputy Chief Signal and Telecom Engineer at Indian Railways, explores how the fourth largest railway network in the world is taking a smart approach in shifting from periodic maintenance to condition-based and predictive maintenance regimes.
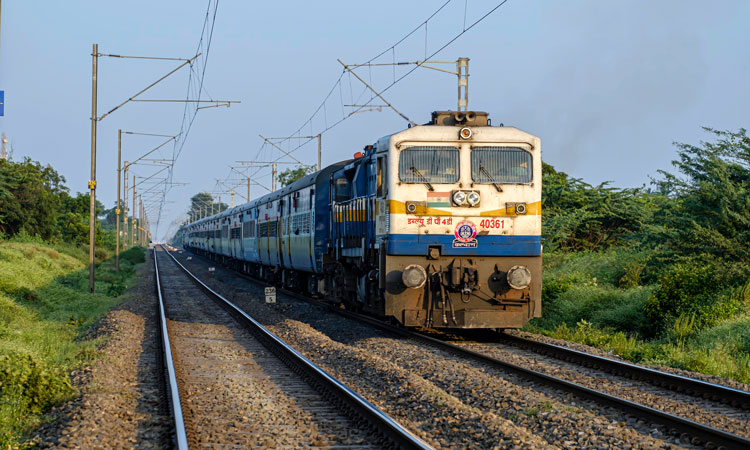
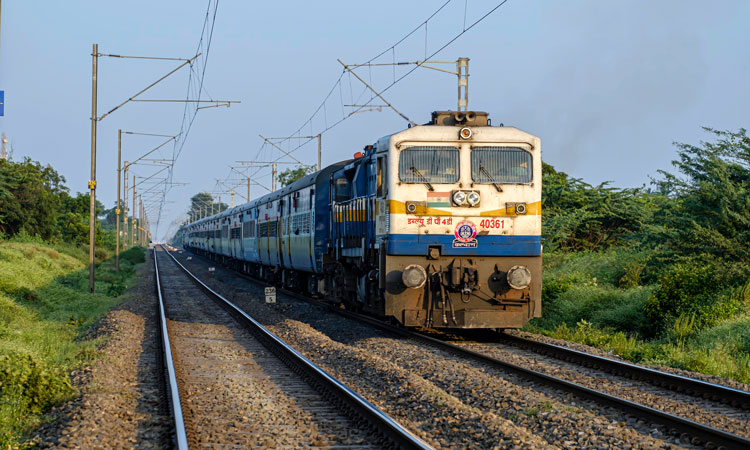
Transport is fundamental to Indian economy and society. With a population of 1.35 billion, the Indian economy greatly depends on the mobility of its citizens. Indian Railways acts as a lifeline for the nation, as it carries almost 13 million passengers every day. The safety of the passengers that Indian Railways serves every day is of paramount importance to the system. Over the years, apart from the regular safety norms that are followed, the network has taken several steps through the innovative use of technology and stepping up the training of its workforce to enhance safety standards. Some of these measures include the replacement of ageing assets, the development of indigenous automatic train protection systems and the use of the latest information and communication technologies (ICT) to make the Indian railway network smarter and to enable much more efficient maintenance.
Shifting asset management trends
Indian Railways is witnessing a shift in asset management regimes, and efforts are in place to switch from periodic maintenance to condition‑based and predictive maintenance. This will certainly help in reducing maintenance overheads on the currently overloaded maintenance staff. Predictive maintenance techniques are designed to help determine the condition of in-service equipment in order to predict when maintenance should be performed. This approach promises cost savings over routine or time-based preventive maintenance, because tasks are performed only when warranted. The main agenda of predictive maintenance is to allow for the convenient scheduling of corrective maintenance and to prevent unexpected equipment failures. By knowing which equipment needs maintenance, maintenance work can be better planned (spare parts, people, etc.). Predictive maintenance has certain advantages – to name a few:
- It helps improve the availability of signalling systems by reducing MTTR (mean time to repair) and increasing MTBF (mean time between failure)
- It reduces maintenance effort
- It brings a collaborative working environment with original equipment manufacturers (OEMs)
- It helps to make data-driven decisions.
The key components of predictive maintenance include:
- Sensing the health parameters of the equipment
- Communicating the health data to a central location
- Analysing this health data to provide actionable decisions
- Dissemination of maintenance decisions to concerned stakeholders.
Data analytics is the field where real value can be drawn. Figure 1 shows how data-driven decisions can lead to actionable insights.
There is a large variety of signalling assets which are installed over the Indian railway network. Consider, for example, a track detection system. The systems for track detection range from conventional DC track circuits – invented in 1872 by W. Robinson (the American inventor, electrical engineer, mechanical engineer and businessman) – to modern axle counters, audio frequency track circuits, balise and RFID tags. Accordingly, the condition monitoring systems have also become more varied and complex, and there is a whole industry working in this area.
Condition monitoring for assets
Figure 2 shows a number of health parameters that can be monitored for different types of signalling assets. Condition monitoring systems for point, signals, integrated power supplies, batteries, axle counters, cables, block instruments and interlocking systems (such as electronic and relay-based interlocking) are required to have effective condition monitoring of all signalling assets. Data loggers are already installed at most signalling installations. Condition monitoring systems can be integrated with data loggers through remote terminal units (RTUs). These RTUs can be connected over a network to send all sensory data to a centralised location, where the data warehouse is then created. All diagnostic and event data collected from all of the discrete monitored devices at a central location provides an opportunity to run various analytic application machine learning capabilities to provide predictive maintenance schedules.
The data repository of diagnostic events‑related information is the foundation of predictive analytics. The more granular data is made available, the better the analytics algorithms are able to find trends, based on which the predictions can be accurate. The algorithms are able to crunch massive volumes of data to find relations and trends between diverse elements, like a relation between vibration and the mechanical wear and tear of the moving parts of a point machine. Thus, to have a truly intelligent and autonomous system, many sensors need to be provided to monitor multiple parameters of the signalling asset.
Since the data collected from such sources will have volume, velocity, variety and veracity, cloud platforms can play a big role in analysing this data and deriving value out of it. Options such as Google Cloud Platform, Amazon Web services, Microsoft Azure and other available COTS products can be explored to analyse these datasets.
Data analytics before condition monitoring
Though there are projects in the pipeline to install a number of sensors to monitor different parameters of signalling gears installed at site, the power of data analytics can be helpful in finding asset failure trends based on past failure history, as well. The predictions done in this manner may not be 100 per cent correct, but definitely provide true information about the upcoming failure with a certain confidence interval.
For organisations that maintain large numbers of assets, it may not be possible to monitor the condition in real-time for every single asset. This requires a lot of money, effort and time. Such investments cannot be planned over a fortnight (for example, the Network Rail- ORBIS programme has been an ongoing initiative since 2012). There is always a possibility of using historical failure data to make estimates for the future. This can help in the better planning of maintenance activities.
Consider, for example, the seasonal variation in failures. Assets such as track circuits, cables and some power equipment are more prone to fail during spring when there is rain, thunder and lightning, resulting in the water logging of tracks etc., which eventually leads to asset failures. Historical failure data can be analysed to run regression models to find a relationship between the level of rainfall at that time of year (week, days etc) and the number or sites of failures, etc. Similarly, other machine learning algorithms (such as time series forecasting) can help in understanding the probability of the failure of a particular asset within a given time interval if it has undergone a specific number of cycles of operation.
These training models will need a considerable amount of data which has been structured properly and accurately maintained over time. Machine learning models can help to find a relationship between different variables and help us predict the outcome with a certain probability and degree of accuracy. Route maintenance teams can smartly plan the maintenance strategy for the route throughout the year and act accordingly. Decisions such as which stations and assets need greater attention, which maintenance personnel needs training etc., if aligned with such data-based models, can yield better results.
A complete asset management system that uses sensory data and captures, monitors and analyses the asset condition data is no doubt the ultimate goal to achieve conditional based maintenance, which reduces maintenance effort and reduces the whole lifecycle costs of assets. But, for organisations which are in the early stages of the transition process of capturing asset performance data on a real-time basis, historical asset data can also be helpful in reducing maintenance efforts by use of proper data analytics tools. This is the power of big data.
Reference
1. Functional Requirements Specification For Predictive Maintenance and Optimization Of Signalling Assets Using IoT & Data Analytics- RailTel Enterprises Limited
Issue
Related topics
Big Data, Digitalisation, Infrastructure Developments, Signalling, Control & Communications, Technology & Software, Track/Infrastructure Maintenance & Engineering