Predictive maintenance: What is the opportunity in rail?
Posted: 13 April 2018 | Simon Stoddart - Altran | No comments yet
Future-gazers in rail imagine a digital world in which the behaviour of every train component and subassembly is captured and recorded. Simon Stoddart, Consultant, Tessella, Altran World Class Center Analytics, discusses how this data version of the train can be used to model and predict future scenarios and identify optimal outcomes.
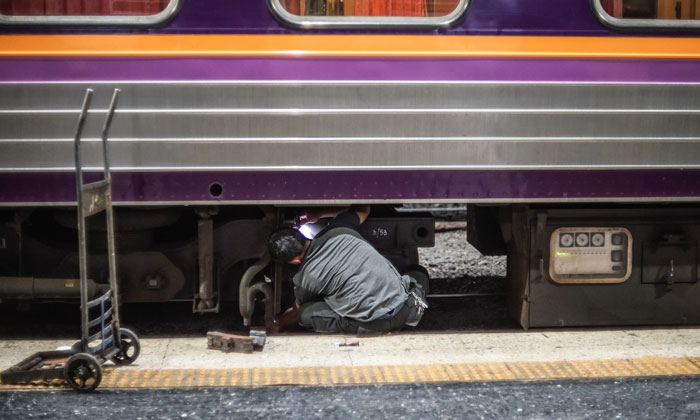
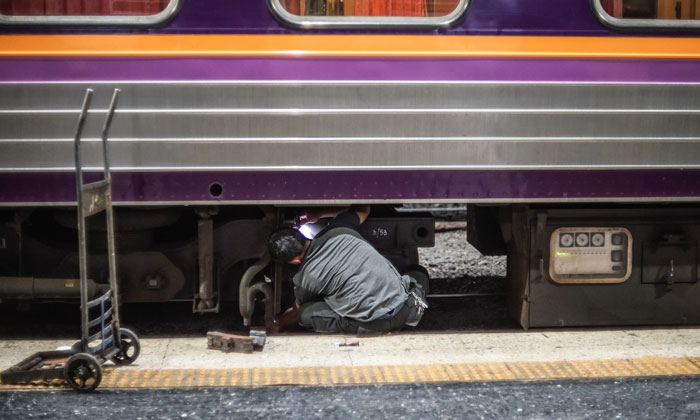
One of the most promising aspects of the rail industry’s digital transformation is predictive maintenance – using data collected on equipment during operation to identify maintenance issues in real time. This means repairs can be properly planned, with the benefits that trains don’t need to be unexpectedly taken out of service for emergency or unnecessary routine maintenance.
There is a lot of hype around this topic and several failed cases. The explosion of IoT sensors and platforms – some overpromising what they can achieve – has created a belief that simply plugging in a few devices will give you all the data you need. A number of poorly planned investments have become costly and fallen short of the expected business transformation.
This should sound a note of caution about buying into hype, but it doesn’t mean predictive maintenance is out of reach. The benefits are there, but they need proper planning and management. As with any promised revolution, there is no magic bullet.
Is predictive maintenance a good rail investment?
Fleets of trains last a long time. Planes and cars are renewed more regularly to take advantage of efficiency improvements from new designs. This is less of a driving factor for trains and the strategic focus tends to be on keeping them in service for as long as possible, to get value out of the considerable initial investment.
That means that technologies enabling predictive maintenance, reducing operating costs and extending a fleet’s lifetime, have the potential to deliver huge financial rewards. However, it also means that older trains currently in service, that were not built for modern connectivity, require investment to be able to do so.
Therefore, there is an important cost-benefit case which must be made ahead of any major investment into predictive maintenance.
Collecting the data for predictive maintenance
Our experience in rail and other sectors is that successful analytics projects start with clarifying the problems that need to be solved and the decisions that are needed to advance forward. Only then should the project consider how to identify the necessary data and the best technology to capture and process it. This helps to work out whether such an investment will be worthwhile.
Two examples of rail operators with similar mid-life commuter fleets that illustrate this spring to mind. Firstly, a company who was installing black box data recorders to meet statutory requirements. Their fleet was experiencing reliability and operational problems, so having committed to the mandatory refit, the company took the opportunity to ask what else it could do to collect data that would improve fleet performance and address the particular problems.
The second company installed the black boxes and connected them with a platform that gave a real-time view of the state of the trains. All captured data was stored but never analysed, until after five years of operation, they decided to look at the historical data to see if there were any useful business insights to be gained.
The first company was able to build up a powerful predictive maintenance programme that reduced maintenance costs and extended the life of its fleet by over a decade. The second found a few useful insights but didn’t have enough of the right data to justify the cost of a predictive maintenance solution.
The right data means the right insights
In our success story, the platform was designed with extensive input from maintenance engineers and other train experts. This helped identify exactly what data was desired.
For example, while capturing data points every second would have met minimum statutory requirements, the team realised that boosting this to every tenth of a second gave a much more useful time resolution for spotting problems in mechanical or electrical systems. They also identified additional sensors that could be combined to provide useful information. Because this was all done at design time, the impact on the refurbishment cost was minimal.
Take a simple example: Train doors. When a door fails, a train must be taken out of service for maintenance. Without data, the technicians in the depot must rely on what the drivers report and since the driving cab is at the front of the train, the driver might not have accurate information. This often means that door faults are not found, the train is sent back out and the problem reoccurs.
In contrast, well-designed data analytics would identify the faulty door and the problem. Changes in door opening times are a precursor to door failure, so it is possible to spot deterioration patterns well before they become a problem that a customer would notice. The maintenance engineer would be informed which door and what to look for.
This approach requires a few things to work: There must be more sensors to detect door position so that door times can be measured and the data would have to be recorded at a high enough time resolution. At one second, a gradual slowing would be easily missed, at one tenth of a second it would be quickly clear when something was going wrong.
Having planned the capture of the right data, it is then relatively straightforward to design suitable algorithms to monitor the doors. Doors can be individually calibrated to measure what is normal and a well-designed algorithm will consider differences between doors and compensate for outliers – someone getting their bag stuck in a closing door, for example. Parameters can be set appropriately.
The principles of this simple example hold for a wide range of challenges. Collect good quality data which is indicative of normal operations, design algorithms which recognise deviations from the normal and match changes to profiles of problems. This enables you to quickly identify when something is going wrong and what it is likely to be – before anyone notices.
Doing more with what you have
In our second example, the data was more limited in scope and resolution. There was also a lack of maintenance records to correlate with the data to identify signatures of common faults. That ruled out predictive maintenance, but it didn’t mean the data was useless.
Indeed, there were some interesting findings. For example, it showed certain trains were performing poorly for months at a time, accelerating up to 50 per cent slower than they should. In one case, we could see that the root cause was activation of traction control on one of two driving vehicles, probably caused by poor calibration of the traction control system leading to erroneous detection of wheel slip. Maintenance records showed that drivers reported the sluggish unit to the depot, however the fault was not corrected for six months, presumably because it only manifested when the train was in motion and in the highest traction notch.
Interesting as such insights were, the development of a system to exploit the data being collected could not be justified. The coverage and time resolution of the data was not enough to support a predictive system and while it was useful for condition monitoring – identifying problems as they occur – this would not have added enough value beyond what was already available.
Where next for predictive maintenance?
The first project described above began more than a decade ago and continues to derive value from predictive maintenance. The fleet, which 10 years ago was believed to be close to retirement, is still going strong. Millions of pounds have been saved in improved reliability, reduced maintenance costs and less frequent purchases of new trains.
Technology has moved on since then. When the project started, data was being sent back in batches up to an hour after being recorded – now multiple sensors can stream data continuously. Data processing is also faster and more sophisticated.
There are also opportunities to use new data capture devices more creatively. Cheap data loggers, which combine smartphone-style accelerometers with a GPS receiver, can reveal a lot about train movements. A recent innovative project used three-axis accelerometers to measure wheel vibrations. Changes in vibration frequency that correlate with vehicle speed could be used to spot vibrations caused by wheel flats, a condition that can damage both the train and the rail.
The data capture and processing technologies have moved on, meaning we have ever more data to play with. But the statistical techniques to turn data into insight, which is where the real value lies, are well understood. To get value, they need to be applied to the right data, with the intended outcome in mind, by people who understand the data and the issue being investigated.
Planning a predictive maintenance programme
Making a success of a predictive maintenance project goes beyond buying out-of-the-box technology or letting a data scientist loose on whatever data you have.
There needs to be an understanding of what you want to achieve. Then you need to work with experts – engineers, maintenance, planners, drivers and data professionals – to understand what data you need to deliver those insights; only then can you work out what technology you need to get that data.
You should only move forward if you are confident in your business case. Refitting a train with new hardware and software costs money. In many cases a well-executed plan can easily justify the investment. But you can only make that assessment if you know what you want to achieve and what it will take to do so.