From track data to asset management
Posted: 10 March 2015 | Matthias Landgraf - Graz University | No comments yet
Reactive rather than preventive maintenance is valuable for in-house as well as outsourced maintenance execution. In order to achieve these goals, the current conditions of the assets, as well as their development over time, have to be considered. Hence, one of the main challenges in railway asset management is to quantify measurement signals in order to implement a preventive, condition-based maintenance and renewal strategy. Matthias Landgraf, Scientific Research Associate at the Institute of Railway Engineering and Transport Economy at Graz University, explains further.
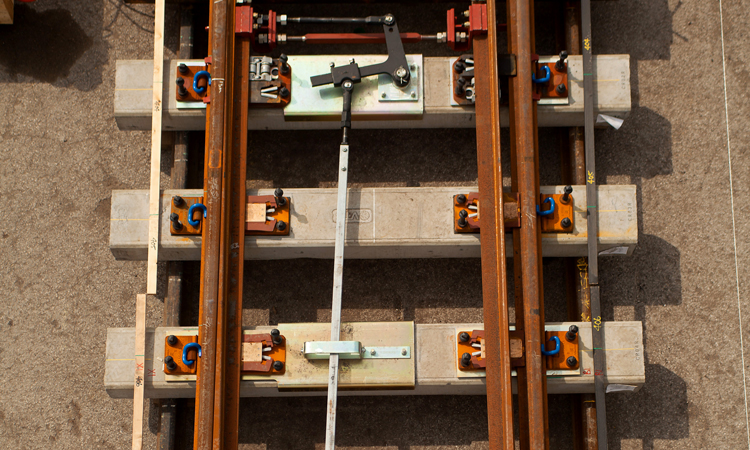
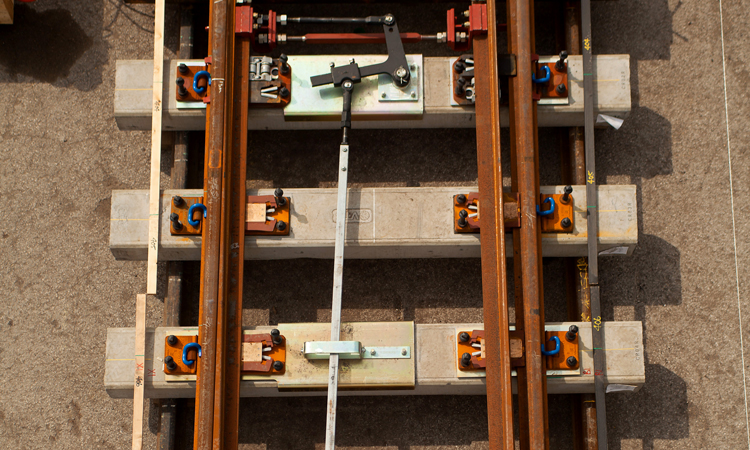
Integrated asset management
Modern asset management has to focus on the following three main parts1:
- The balance between maintenance, renewal and enhancement
- The link between infrastructure managers and contracting companies
- The combination of the strategy and its implementation based on evidence-based decision making.
The Institute of Railway Engineering and Transport Economy at Graz University of Technology has been working on the field of life-cycle costs (LCC) since 1996. Based thereupon, asset management has been developed in close cooperation with the Austrian Federal Railways (ÖBB). The renewal of track is one of the main cost drivers in track asset management. Therefore, it is crucial to identify the ideal point in time for reinvestment. Within the ÖBB, this process is called Life Cycle Management2 and is supported by Graz University of Technology. From an economic point-of-view, depreciation decreases with increasing service life. However, costs of maintenance and consequently costs of operational hindrances grow. As a result the annuities reach their minimum before the influence of increasing maintenance costs grow too big. Therefore, the ideal point in time for track renewal (economic service life) is defined by the minimum of annuities.
In this process it is essential to evaluate the track’s condition in order to predict necessary maintenance measures. There are two possible approaches: (1) Use the experience of track staff and historic records of maintenance actions for different boundary conditions, or (2) establish a sophisticated track measurement evaluation that describes the current track condition and its future maintenance needs by an analysis of the deterioration of track quality over time. We chose to combine both aforementioned approaches. The process of Life Cycle Management was initiated by using historic information and experience while more and more sophisticated evaluations coming from track data analysis are implemented simultaneously.
Data warehouse
Over recent years, recording cars have been used to measure different types of signals. Consequently, the amount of measurement data has increased dramatically. On the one hand, this could possibly allow for a better evaluation of the current track condition, but on the other hand the complexity of the analysis increases significantly. Thus a precondition for the analysis of track measurement data is proper data management. Since 2003, a data warehouse has been established in close cooperation with the ÖBB. Currently, this data warehouse contains track information of more than 4,000 track kilometres of Austria’s main network with information on cross-sections every 5m. Up to now, the data warehouse provides linked information concerning the following three main points: (1) general asset information (type and age of sleepers and rails, traffic volume and executed maintenance actions); (2) a complete history of measurement signals since 2001 as provided by the Austrian measurement coach; and (3) evaluations from ground-penetrating radar and the Geotechnical Track Report, evaluating substructure and drainage conditions from in-situ inspections. Combining all this, condition-based monitoring for the main network in Austria can be carried out. In addition to the available measurement data, a regression model with exponential deterioration functions is carried out on vertical standard deviation as well as on a generated velocity-dependent track quality figure.
The behaviour of track over time can be expressed based on simple technical experience: a good track behaves well, while a poor one deteriorates faster. In other words, the deterioration of track geometry depends on the present quality level. Mathematically, this leads to the following equation2..
…where the track quality Q at any point in time can be calculated using Qn as the initial quality after relaying or executing a maintenance measure, while bn describes the deterioration rate within this particular deterioration period. A high initial track quality can be ensured by a proper investment. Additionally, the high initial quality can be used to achieve a long service life through proper maintenance actions. Short-term cost reductions by reducing maintenance costs will therefore cause a reduction of service life and thus dramatically increase long-term costs. Sustainable asset management therefore needs to be a strategy that comprises both investment and maintenance.
Track data evaluation
Apart from exploring the behaviour of commonly used measurement signals, we put a lot of effort into research on innovative quality values. Besides the aforementioned velocity independent quality, the following two main quality figures to describe the component-specific asset condition have been established within the last years: (1) a modified standard deviation of track gauge4 in order to describe the condition of fastenings, railpads and sleepers, and (2) fractal analysis of vertical track geometry in order to describe the condition of ballast, formation layer and subsoil.
Failures of ballast, formation layer and subsoil are the main reasons for track renewal, which founds the necessity of evaluating their conditions. Moreover, former research2 showed that insufficient substructure quality and malfunctioned water drainage increase maintenance demands (tamping, ballast cleaning) and decrease the track’s service life (necessity of frequent interventions). Water problems may cause slow orders (summer: deflection; winter: frost buckling) which leads to costs of operational hindrances and thus considerably increase LCC. Therefore, modern railway management needs to provide a methodology which enables a classification of the current substructure condition.
Fractal analyses
We evaluate ballast, formation layer and subsoil conditions with fractal analysis. Hence fractal analysis of vertical track geometry was applied on all 4,000km included in the data warehouse. This analysis methodology is based on the fact that vertical track geometry can be derived from a sum of harmonic irregularities with different wavelengths and their amplitudes. Mean values or standard deviations, as commonly used, focus on the amplitude of defects within the alignment, but neglect the wavelength – for instance, the characteristic of a failure. Fractal analysis of vertical track geometry describes the characteristic of a failure by delivering expressive and informative quality figures in a comprehensible way5. The so-called Modified Divider Length Method6 enables us to split vertical deflections into their different ranges of wavelengths. We divide the wavelength ranges into short-waved (wavelengths: 0m-3m), mid-waved (3m-25m) and long-waved (25m-70m) errors. Deflections in vertical track geometry, that are caused by ballast problems are more likely to occur within the mid-waved range while deflections caused by insufficient substructure conditions are expected to appear as wavelengths larger than 25m. Since the short-waved errors are not considered to be linked to ballast bed or subsoil problems they are not discussed further within this paper. Mid-waved as well as the long-waved ranges are described within EN1348-57 as wavelength ranges D1 and D2. This approach of chosen wavelength ranges appears to be valid for the Austrian speed ranges, while on high-speed lines (more than 250km/h) the wavelength range D3 might be taken into consideration as well. For a fractal analysis of vertical track geometry only the raw measurement signal of the longitudinal level for vertical track geometry is required. This can be delivered by any state-of-the-art measurement coach as used from most of the railway infrastructure companies.
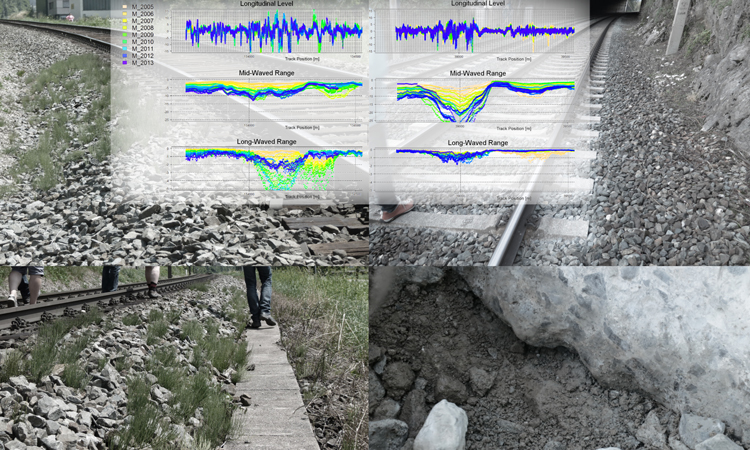
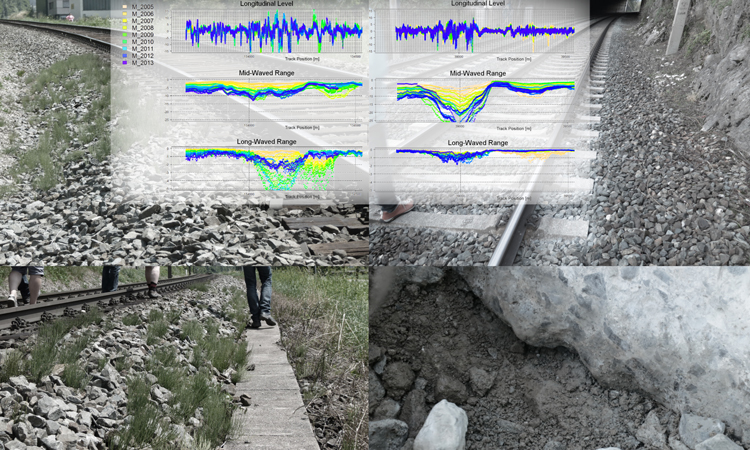
Figure 1: Fractal analyses compared to in-situ conditions
Figure 1 shows the two possible evaluation results of fractal analysis and the corresponding track condition during an in-situ inspection. On the left side the deflections of the longitudinal level occur mostly in the long-waved range, while the mid-waved dimensions retain an adequate quality level. This leads to the assumption that a problem is present in the substructure area while the ballast bed (still) provides adequate quality behaviour. This is precisely what can be seen in the image on the left side: The upper part of the image shows a blocked dewatering system. Consequently, any water will pass through the track. Unfortunately, as shown, a concrete cable funnel hinders the water from draining. Thus, the water is kept under the ballast bed, which leads to a decrease in loading capacity of the formation layer and thus the subsoil. Accordingly the evaluations of fractal analysis display long-waved irregularities within this section. Beyond that, the visible vegetation in the ballast bed also indicates a very moist area which again fits the assumption that the water is not drained properly.
It is obvious that the errors within the longitudinal level occur within the mid-waved dimension. This leads to the assumption that the ballast is in poor condition. The top right picture shows white spots around the sleepers which results from ballast crushed due to overstress. The lower image displays that there is already a very large amount of fine-grained material (crushed ballast) in the ballast bed and also the bottom edge of the sleeper is worn out resulting in an inadequate load transfer.
These two examples show that fractal analysis is a suitable tool for monitoring the track’s condition concerning ballast and substructure quality. Furthermore, it can be easily implemented with standard measurement data from recording cars as used from many railway companies all over the world. Currently, the network-wide validation process is finished and showed promising results. In the next step, this methodology will be implemented for the entire Austrian network to classify ballast and substructure condition.
Conclusion
Asset monitoring and preventive maintenance planning require sophisticated evaluations of track measurement signals. In addition to the exploration of commonly used measurement signals, the Institute of Railway Engineering and Transport Economy at Graz University of Technology established fractal analysis of vertical track geometry to provide component specific condition monitoring. With fractal analysis a distinction can be made whether a failure in vertical track geometry is caused by an insufficient ballast bed or an insufficient substructure quality. Consequently, the current asset condition can be evaluated and the remaining service life can be estimated more precisely. Furthermore, sections requiring subsoil rehabilitation within an upcoming track renewal can be identified. Thus, the point in time for renewal as well as its extension can be estimated at an early stage. This leads to a more precise planning process and, consequently, to lower costs. Moreover, the knowledge of technical requirements in advance leads to a more stable budget plan.
References
- Rail System and Communciations Department (2011). Guidelines for the Application of Asset Management in Railways Infrastructure Organisations. Paris: International Union of Railways (UIC).
- Veit P. (2007). Track Quality – Luxus or Necessity ? RTR Special, pp. 1-5
- Hansmann F., Marschnig S., Landgraf M. (2013) GleisPROPHET – next step to sustainability. Proceedings, World Congress on Railway Research.
- Hansmann, F. (2015) Innovative Messdatenanalyse – ein Beitrag für ein nachhaltiges Anlagenanagement Gleis. PHD-Thesis, Graz University of Technology
- Landgraf M., Hansmann F., Marschnig S. (2014) Track Geometry and Substructure Condition – a provable Correlation? GEORAIL 2014, IFSTTAR, pp. 623-632
- Mandelbrot B.B. (1989). Fractal Geometry : What is it, and what does it do ? Proceedings, Royal Society A 423, pp. 3-16
- CEN/TC 256, 2005. Railway applications- Track geometry quality EN 13848-Part 5: Geometric quality assessment, Vienna: Austrian Standards Institute.