Unlocking smart turnouts through the digitalisation of rail
Posted: 3 September 2021 | Robert Bernerstätter | No comments yet
Dr. Robert Bernerstätter, Data Scientist for R&D and Engineering in Diagnostics & Monitoring Technologies for Infrastructure at voestalpine Signaling Austria GmbH, writes of the potential that lies in smart turnouts, to increase availability and, through the use of AI, predict maintenance needs and in turn, reduce costs.
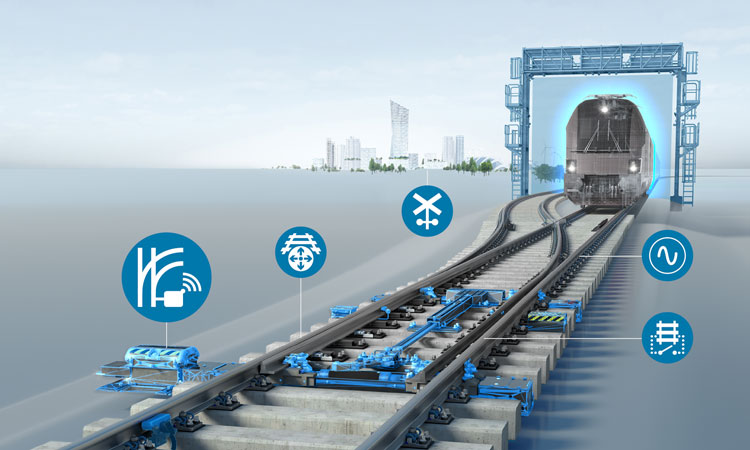
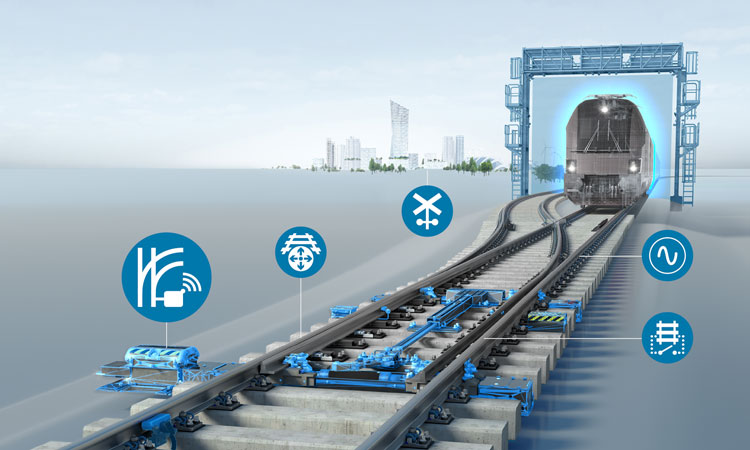
Diagnostic and Monitoring Technologies for Infrastructure – Digital ‘Performance on Track®’
Digitalisation is an essential building block for ensuring and increasing the availability and efficiency of railway infrastructure. The turnout, as an interface between track and signalling technology, has long been a focal element of data acquisition and monitoring. It is therefore predestined to be further developed into a smart device with the corresponding components. This not only increases availability, but also reduces lifecycle costs (LCC) in the long-term.
Why is there a need for smart components in railway?
Despite initiatives to invest in the expansion of the railway infrastructure, the railway system is in competition with other modes of transport. Efficiency and competitiveness must be further increased, expressed in the reduction of LCC. An essential component of LCC is operating costs caused by maintenance. The digital transformation is a central key to increasing availability and thus reducing LCC. However, it is only just beginning in the area of railway infrastructure.1
Turnouts, as one of the key elements of the track, essentially determine its safety and availability. For this reason, acute turnout failures that cannot currently be predicted are among the top 10 life-cycle-cost drivers for railways. Switches account for approximately one per cent of the total investment costs for new route construction, but 25 per cent of the maintenance costs of a route.2
Maintenance costs are strongly influenced by the ability to plan maintenance measures. Direct maintenance costs result from maintenance activity, whereas indirect maintenance costs are caused by a turnout malfunction and have a disproportionately higher share in acute/unplanned malfunctions than in planned maintenance.
Digitalisation is an essential building block for ensuring and increasing the availability and efficiency of railway infrastructure.
The aim must be to turn unplanned failures into planned maintenance, which has no or only minimal influence on the operation of the train and therefore has lower indirect maintenance costs and a lower impact on the LCC. Many acute failures announce themselves in advance by measurable changes in the behaviour in the turnout component or can be predicted by simulations and the use of load data. A small increase in investment costs, due to the sensor equipment of the turnout, is countered by a larger reduction in operating costs.
What characterises a smart turnout?
Although possible and available, sensors and so-called turnout diagnosis systems are yet not standard. The analyses are limited to acute malfunctions in the point machine. The aim is to avoid unplanned and costly downtimes and maintenance.3
Functionality
A smart turnout is aware of its environment and condition, can provide information about it and ideally react to it. Artificial intelligence (AI) in the broadest sense is an essential component to give smart turnouts this ‘awareness’. A smart turnout collects relevant data, processes them locally, if necessary, and transmits the results or raw data. The transmitted data are further processed at a central location.
Components of a smart turnout
Sensor packages are required to record the environmental parameters and their own condition, which provide relevant data. They measure the influencing parameters in order to identify the most important failure modes of the turnout components. The smart turnout is equipped with a data transmission device for data transfer. Since it is not absolutely necessary to transmit the raw data, the smart turnout should also be able to process and evaluate the data decentrally.
The software that processes the data is another essential factor of a smart turnout. It either pre‑processes the data decentrally directly at the device, or it is processed centrally. The software comprises the classic tasks of data connection, retrieval and storage and is used to integrate the analysis component and the AI into the smart turnout system.
Application of AI
The analysis component can be structured in four successive stages.4 The descriptive level allows visual analyses by displaying the measurements in graphs, key figures or similar. The diagnostic stage provides information on the condition of the turnout. Failure modes of the turnout components are compared with the measurements and more complex machine learning algorithms may already be used. The prognostic level makes a statement about the remaining useful life (RUL) of the component and at the prescription level, the system supports the user in making decisions. Recommendations for action are given.
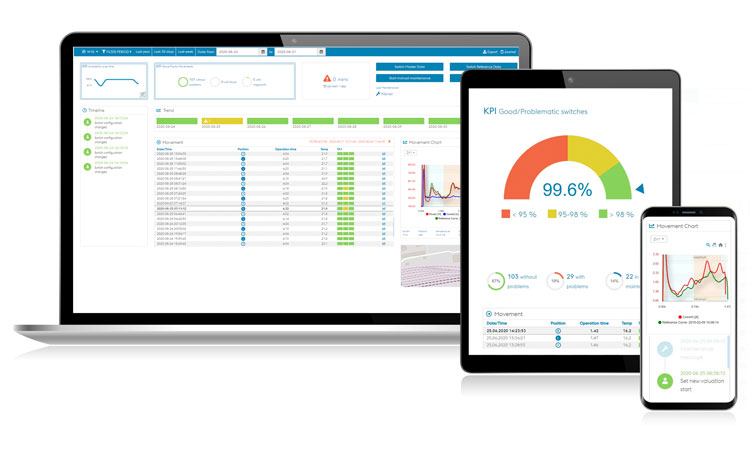
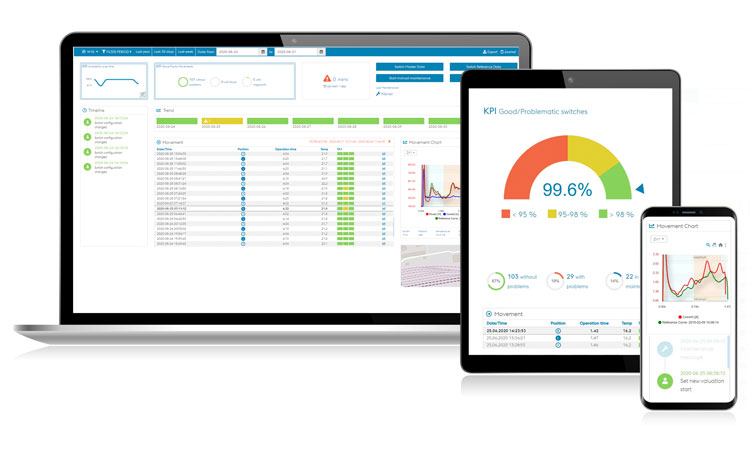
Visualisation and analysis example of the diagnostic and monitoring system ROADMASTER® for condition and future oriented maintenance.
Challenges in the use of AI
The field of application of switch condition monitoring systems (e.g. ROADMASTER® SCM) can be ascribed to predictive maintenance. The aim is to detect the degradation of the turnout and to predict the time of failure early and reliably. The challenges have, in most cases, to do with the data – it has to be suitable for the goal of the analysis.
Missing learning cases
The railway industry is confronted with the paradoxical problem that the turnout is designed to be very reliable and in addition the maintenance regime usually provides for tight service intervals. This is intended to prevent failures. This is good for the operation of the infrastructure, but bad for the development of diagnostic and prognosis systems. This is known as the phenomenon of unbalanced datasets. A disproportionately large number of good cases is matched by a negligible number of failures. Algorithms that are supposed to learn the differences from large amounts of data face problems here.4
Lack of alignment in digitalisation
Digitalisation development is proceeding at different speeds in some areas. While automated switch monitoring is being increasingly digitalised, digitalisation has hardly started for the manual activity of turnout maintenance. This leads to an increasing discrepancy and a tension in two areas that are supposed to benefit from each other, because automated systems require reliable input about manually performed activities at the turnout in the field.
Improper measurements
In order to correctly identify failure modes and to predict failures at an early stage, the appropriate physical quantities must be measured to allow conclusions to be drawn about degradation. This requires domain knowledge from the design and diagnosis of turnout.
Lack of domain knowledge
Experts’ knowledge of the turnout system is an essential success factor in the evolution towards the smart turnout. Without knowing what is to be measured and how the results are to be interpreted, the smart turnout is all the more difficult to implement.
Conclusion
The smart turnout will drive forward the digitalisation of the railway infrastructure. The combination of hardware for recording the condition of the turnout and its environment, with software and artificial intelligence is essential in this process. A key factor that should not be underestimated, however, is the domain knowledge about the railway infrastructure system in general and the turnout in particular, which gives system providers a decisive advantage in fulfilling the wishes of their customers according to their needs.
References:
1. Scordamaglia, D.: Digitalisation in railway transport-A lever to improve rail competitiveness, European Parliament Research Service, 2019
2. Fendrich, L.; Fengler, W.: Handbuch der Eisenbahninfrastruktur, Springer Vieweg, 2013
3. Fritz, Ch.: Intelligenter Weichenantrieb-Intelligent Point Machines, SIGNAL+DRAHT 10/2018
4. Famurewa, S.; Zhang, L., Asplund, M.: Maintenance analytics for railway infrastructure decision support, Journal of Quality and Maintenance Engineering 23-3/2017.
Issue
Related topics
Artificial Intelligence (AI), Big Data, Digitalisation, Technology & Software, Track Systems, Track/Infrastructure Maintenance & Engineering