Simulation of interrelated delay effects and the need for international collaboration
Posted: 2 September 2021 | Gerald Schinagl, Peter Blauensteiner | No comments yet
Gerald Schinagl, Digital Innovation Manager at ÖBB BCC GmbH and Peter Blauensteiner, Programme Manager of Strategic Capacity Management at ÖBB Personenverkehr AG, write how a predictive, rather than a reactive, approach to delay management could transform rail timetables.
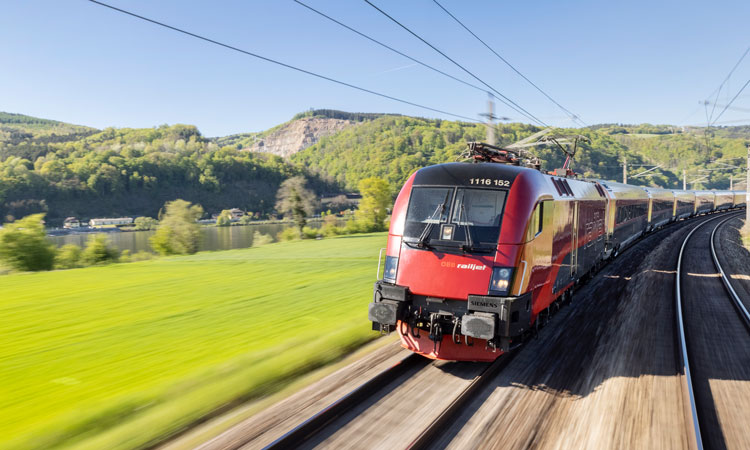
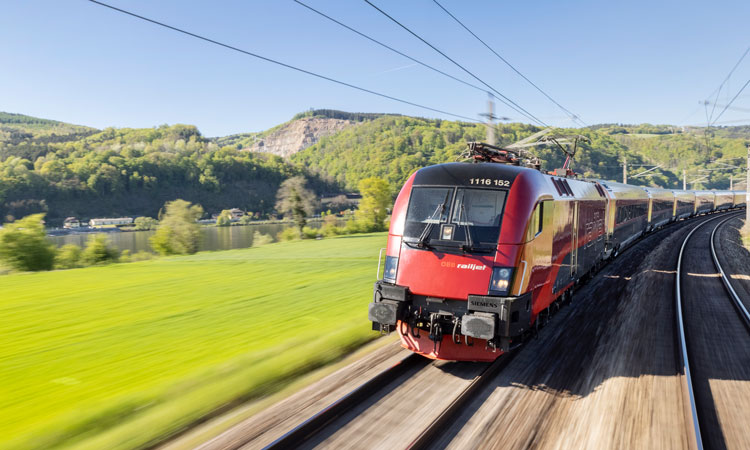
Credit: ÖBB/Harald Eisenberger
Delay management in the European railway system currently largely consists of drawing appropriate conclusions from the delays that have already occurred (characterised by assigned delay codes), deriving possible causes of the observed effects and thus resulting in future improvement. The practice of predicting preventable delays and taking immediate action is not yet the default way of handling issues. However, it would lead to better results than the current, reactive approach.
Establish preventative processes
As part of the strategic capacity management programme in ÖBB Personenverkehr AG, for the first time, we made an attempt to determine whether it is possible to carry out this kind of prognosis with sufficient accuracy. Furthermore, we wanted to establish preventive processes based on the simulation-outcome. The objective for the trial, which we are reporting on in this article, was to use a given timetable, a known rail network, planned and reported influencing factors (for example, construction sites and slow-movement areas). The trial also used, generally, expected influences (such as exceeding the stopping time and delays from neighbouring countries) as variables in a calculation and simulation logic to estimate the resulting overall delays.
After an initial evaluation, we decided to utilise agent-based modelling, as this method matches the nature of the task, but also enables a relatively resource-saving simulation process. This simulation considers each train as an individual mobile agent that moves through a rail network (limiting the degrees of freedom in movement) according to a timetable and tries to comply with this requirement as far as possible. These mobile agents are affected by the delay inputs, and the mobile agents influence each other on the scarce infrastructure.
Understanding delays
The next steps in the further development of this prognostic model are to improve the breadth and depth of the data-foundation.
These reasons for the delays are geographically allocated to the underlying route and then assigned to the trains that pass them (virtually). Depending on the influence of the delay, it can be a fixed value (within a statistical variability) or a calculated value, for instance in the case of a speed limitation, that is used. Moreover, each delayed train consumes the provided timetable reserves on the route until the train is on time again. For this simulation, a position in the transport network (more precisely in a route section) in which each train is located, is calculated for each point in the simulated timeframe. Based on this information, the mutual influence of trains is derived. This can lead to blockades on single-track lines (waiting for the opposite train). Moreover, one can calculate an approximate minimum distance between two trains if there is only one track in each direction.
In addition to the technical solution that is described later in this article, we also had to develop our own classification formula for delay influences according to their influencing characteristics as part of this project. This is necessary because the delay codes are suitable for the post-ex analysis and not for cause-and-effect assessments.
Implementing the prototype
We ultimately implemented this prototype as a numerical simulation using a relational database and classic programme code. We opted for this technically rather simple approach, because the environment, due to rigid specifications such as timetable and the rail network, is already limited to such an extent that the frameworks for agent‑based modelling can only show their strengths to a limited extent. Coding these limitations into typical frameworks generates a lot of effort, which is not worth the benefits. However, we have also dispensed with specialised solutions for simulating rail traffic, as we are dealing with heterogeneous output data in different granularities, and most of these tools require a physically exact mapping of the route, which is a laborious task. Furthermore, we wanted to carry out this prototype, which should clarify whether the technical-methodical approach is even suitable without being tied to manufacturers or licenses.
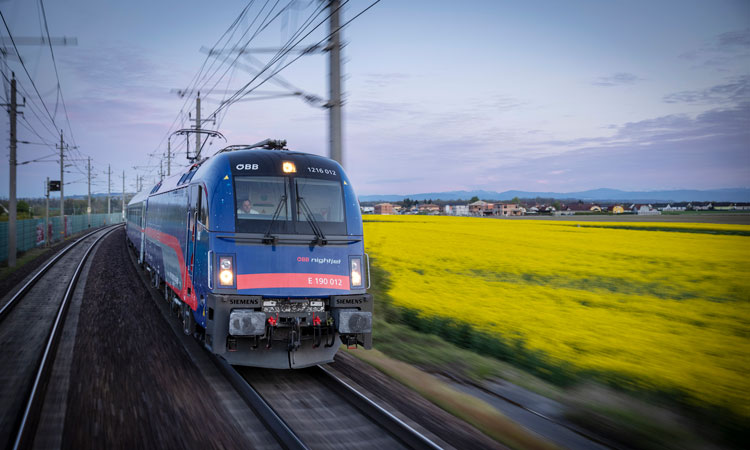
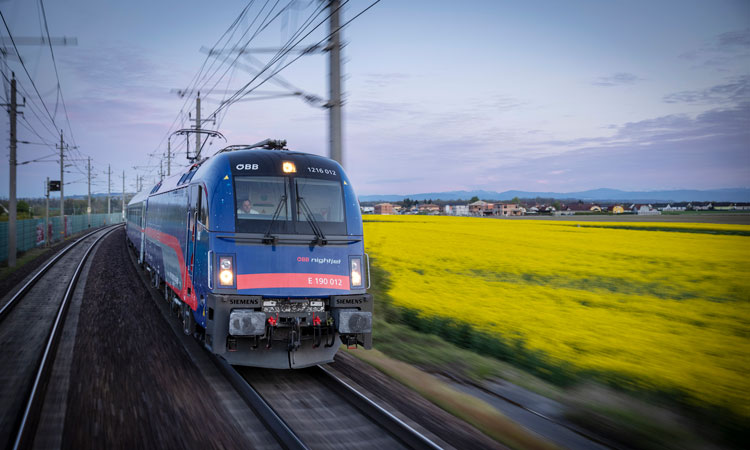
Credit: ÖBB/Harald Eisenberger
Summarising the agent-based model
In conclusion, the chosen method of an agent-based model works very well, provides new insights into the development and dynamics of delays for different scenarios or initial conditions, and thus has the potential to support the preventive avoidance of delays due to suitable measures in the operational processes. From a technical point of view, the challenges, especially when using an in-memory database, are not too demanding and a simulation of the entire Austrian rail traffic can be made with common hardware (without the need for GPUs).
We see potential in a higher parallelisation (better use of the many processor cores). However, this is more complex to implement due to the mutual dependencies and only makes sense if real-time simulations are required.
The need for data science
As always with simulation projects, the challenge lies in the data, its availability and suitability for this purpose. The required data is usually available, but first must be prepared for usage in the simulation. Otherwise, it may lack necessary details. This is the typical challenge of ‘downstream’ data usage, since this data was originally collected for a different purpose. The need for data science also quickly becomes apparent, not only to calculate assumed and derived influences, but also to realistically assign them (for example, daily dependencies and seasonalities) to the agents.
Impact of COVID-19
One must also consider that the effects of COVID-19 made it difficult to compare simulation and reality. Typically, one proceeds with simulations in a way that one simulates the next few days and then compares it with the reality that has occurred. In our case, however, this situation changed continuously (Austria went into a lockdown during the test period) and other framework conditions (emergency allocation of services, timetable changes, etc.) were in a constant flux. Given this situation, an approximate suitability of the simulation model could be proven, but a detailed analysis is only possible when the regular schedules and standard conditions have returned.
Unexpected challenges
Even without the pandemic, the comparison of simulation and delay coding on the level of a single train turned out to be more challenging than expected. This is due to the different acquisition paths of the outcomes or the process of data creation which, when comparing these two information sources, results in a lack of clarity. Sometimes even the smallest unplanned delays – that have not been included in the simulation – are enough to produce massively deviating results.
A large uncertainty in the estimation of delays in international traffic is the delayed border crossings, for which there has not yet been a sufficiently precise data exchange. An estimate, which is ideally acquired from a similar simulation activity in neighbouring countries, would be helpful to facilitate a more accurate simulation. An international and standardised exchange of simulation results via an electronic interface (which differs from reported delays or announced limitations) would be beneficial for European rail traffic.
Next steps
The next steps in the further development of this prognostic model are to improve the breadth and depth of the data-foundation. Additionally, one needs to increasingly incorporate the logic of data science and other influences, which are more subject to an estimate and derivation from historical data and patterns, into the model.