Using prognostics and health management to maintain railway infrastructure
Posted: 5 October 2018 | Dr. Madhav Mishra | No comments yet
Railways are important transportation systems within society, and the pressure on their services has been increasing due to a rise in adoption, population and traffic flow. Dr. Madhav Mishra, Senior Researcher at Luleå University of Technology, Sweden, discusses a maintenance technique that could help managers in meeting this demand.
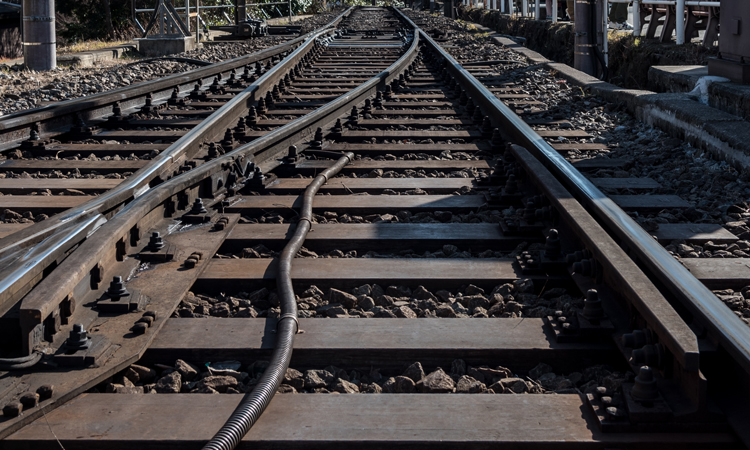
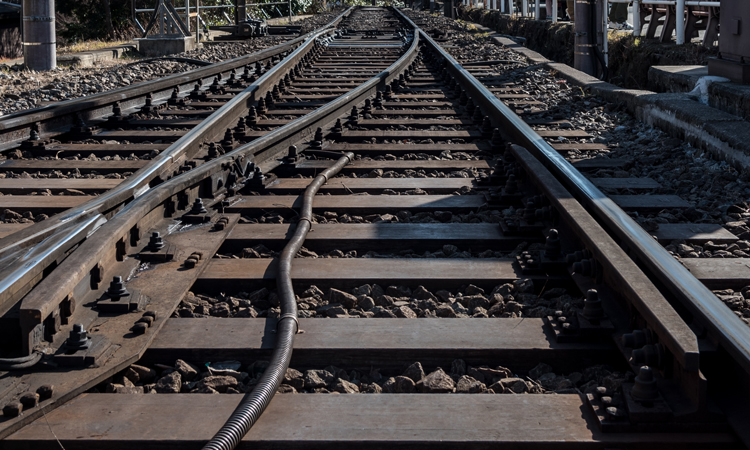
In response to the increased demand for rail, infrastructure managers (IMs) must reduce the occurrence of unexpected failures, enhance train capacities and reduce overall costs. Effective maintenance planning requires prognostic capabilities to avoid failure and provide corrective solutions for operation and maintenance issues.
A railway track consists of many different components, and one major aspect related to maintaining the line is the conservation and restoration of the track geometry, especially for ballasted tracks. This helps avoid increased wear and therefore reduce the risk of derailment. Track geometry degrades over time, dependent on various environmental aspects and traffic load. The traffic load is cyclical in nature, and the degradation can be described as a combination of two processes: The settlement process (which is mainly driven by the traffic load between maintenance actions) and a degradation process (characterised by restorability).
The servicing of railway tracks is performed by planning and executing a variety of maintenance actions. For ballasted tracks, which currently represent the majority of railway networks, tamping is an important action that aims to restore the designed geometric shape of a track. Failing to ensure this could result in safety and comfort issues and consequently speed restrictions or the line closures.
To this end, there is a need to develop prognostic and health management (PHM) technology for railway track geometry – to facilitate better operation and maintenance decision making.
PHM can be used to improve asset utilisation and the length of life by predicting the future condition of the asset, which will suggest corrective actions. For example, it could estimate the remaining useful life (RUL). The main benefits of prognostics lie in anticipating future failures to increase uptime, implementing dynamic maintenance planning to decrease costs and reducing energy consumption. Another major question in maintenance planning is when to act, or in other words, how long intervention can be postponed. By performing an end-of-life (EOL), in this case EOL is defined as failure at the next tamping action, estimation at time T and the risk of failure due to an excessive delay in intervention (for example, until time T+t) can be measured as the integral of the EOL probability density function (pdf) from – ∞ to T+t.
In railway infrastructure, the track geometry must be maintained to ensure track safety and functionality. Therefore, the track degradation in ballasted railway track systems must be measured on a regular basis to determine when tracks should be tamped. Tamping aims to restore the track geometry to its original state; ensuring an efficient, comfortable and safe transportation system. To minimise the disruption caused by tamping, this maintenance action must be planned in advance. Track degradation forecasts derived from regression methods are used to predict when the standard deviation of a specific track section will exceed a predefined maintenance or safety limit.
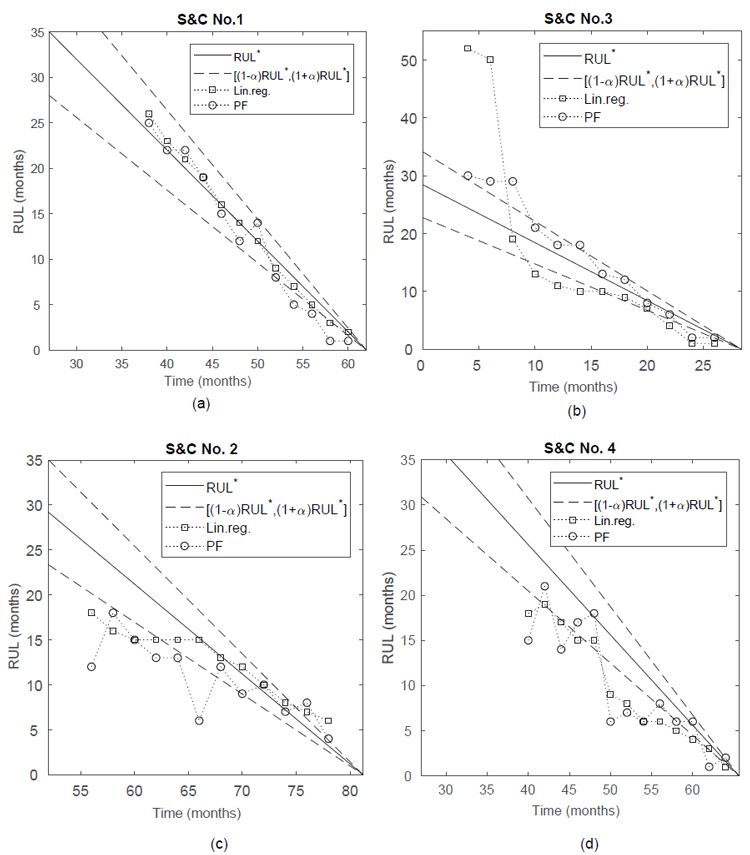
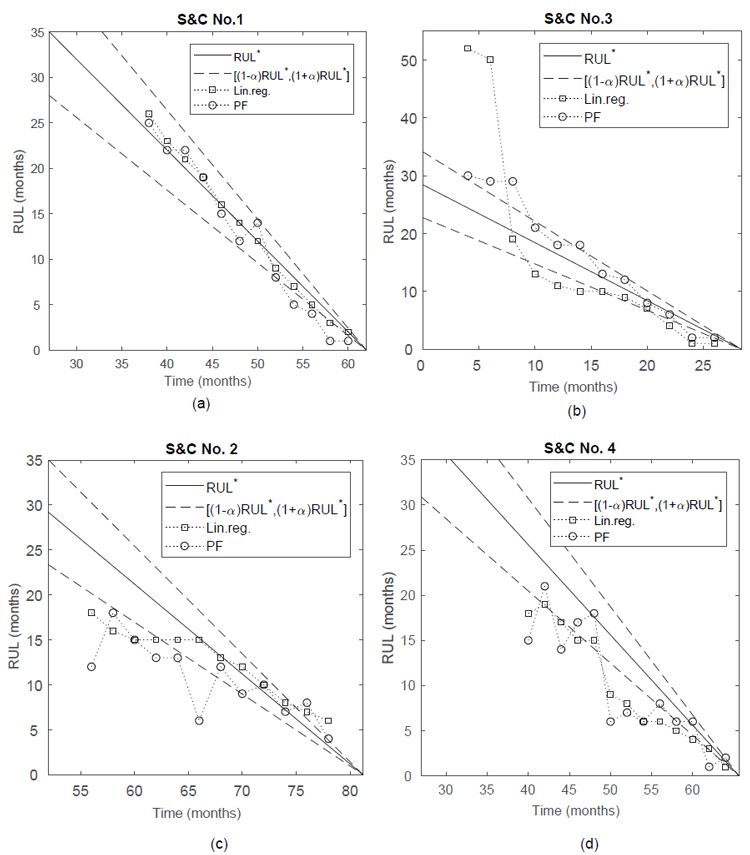
Figure 1: Comparison of the RUL predictions from regression and state estimation using particle filters for S&Cs No.1,2,3&4. The figures show the deviation of the prognostic compared to the elapsed time. The allowable error bound value α is set to 0.2. The slope of the curve represents the remaining time to ‘failure’ (RUL) for each point in time1
Many general methods exist for predicting the RULs of systems and components. The most common prognosis methods are physics-based approaches, which rely on mathematical formulations based on physical phenomena or principles, and data-driven approaches.
Today, many railway IMs are focusing on risk-based maintenance planning1,2. This can be addressed through the proposal of an alternative method for track geometry prognostics that offers probabilistic predictions to facilitating risk assessments and avoiding uncertainties due to noise (since sigma – the standard deviation – is noisy and sometimes difficult to obtain accurate predictions using linear regression).
To be precise, we have proposed a particle-filter-based prognostic approach for railway track degradation at railway switches. Our work is currently published in Mechanical Systems and Signal Processing, MSSP1 and the purpose is to predict when railway track geometric degradation will reach the maintenance limit. The main questions here are how to incorporate probabilistic predictions and how to facilitate a risk-based maintenance decision process.
Our approach has been demonstrated on a set of standard deviations of measured longitudinal track geometries for four railway switches. The distribution should preferably describe the inherent uncertainty of the degradation process and future operations as well as the errors associated with the utilised prediction techniques. Then, prognostic decisions can be made on the basis of a probabilistic forecast rather than a specific deterministic value without any associated probability, as can be seen by the RUL results in Figure 1.
In summary, we can conclude that the particle-filter-based prognostic approach for railway track geometry incorporates the uncertainties in train loads due to different traffic situations. This approach generates probabilistic results that can facilitate risk-based decision-making, and when limited data is available, it uses a degradation model. The results of the proposed method are compared with the results of an existing approach1.
References
- M. Mishra, J. Odelius, A. Thaduri, A. Nissen and M. Rantatalo; “Particle filter-based prognostic approach for railway track geometry” Published in Mechanical systems and signal processing, 96, 2017, p. 226-238 https://doi.org/10.1016/j.ymssp.2017.04.010
- M. Mishra; ‘Prognostics and Health Management of Engineering Systems for Operation and Maintenance Optimisation’ PhD dissertation, Luleå, 2018. (Doctoral thesis / Luleå University of Technology). http://urn.kb.se/resolve?urn=urn:nbn:se:ltu:diva-68345