Aerial analytics: Laying the tracks of a new intelligence
Posted: 26 August 2020 | Pradeep Sukumaran | No comments yet
Pradeep Sukumaran, Vice President – Business Strategy and Marketing at Ignitarium, explains how the company’s rail track defect detection solution – which uses aerial vision analytics – can use intelligent data to spot rail line faults without disrupting train schedules.
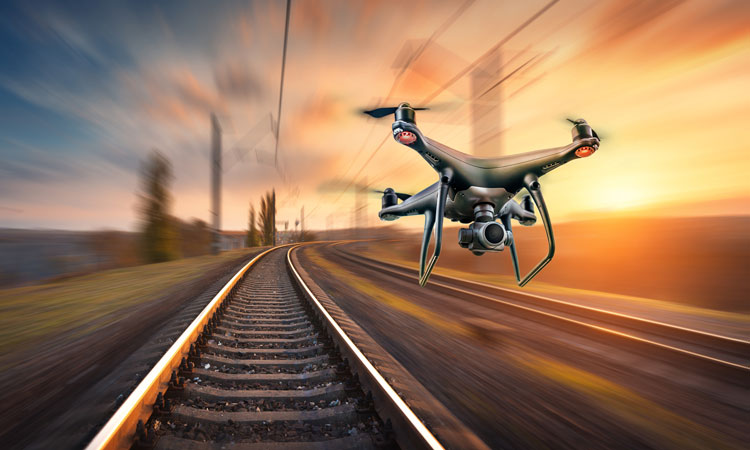
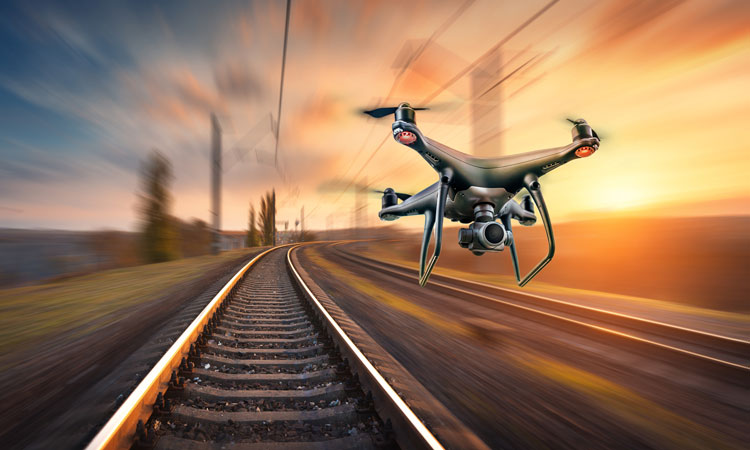
Remember your first train ride? Depending on where in the world you grew up and when, the memory differs. Mine is from the 1980s in India: a trip back to my ancestral home in Kerala. From the minute the engine blows its resoundingly loud whistle, there’s magic in the air. You scramble to your seat alongside strangers soon to be friends. And while you gaze out through the window into the expanse as the train rolls along, you can’t help but think that this box on wheels is a slice of home. As warm as it gets, and just as safe.
That’s the idealist in me talking. The pragmatist pulls me in another direction: to peek closer at the 123,542km of rail tracks in India alone. The U.S. is 202,500km, and the European Union spans 225,625km of rail: almost five times the Earth’s equatorial length. When you’re dealing with such a large network, the statistics are overwhelming, and you’re bound to have room for error. The story is the same for everyone in the global railroad maintenance industry: they can’t man every crossing or regularly inspect every inch of every track, can they?
Gauging the state-of-the-art safety
Most accidents are avoidable, the common causes being derailments, level crossing accidents, collisions, and other hazards such as fire. Great Britain witnessed 517 train accidents in 2018-2019, while the U.S. reported 2,200 incidents in 2018. Before I paint too grim a picture, on the bright side, all these numbers are among the lowest they’ve ever been since the inception of the railroad over two centuries ago. Today, the focus is on enhancing surveillance to improve anomaly detection. The outcome we all desire is better hazard prevention and control. We’re getting there. In fact, the U.S. numbers in 2018 were 92 per cent lower than the 12,000 incidents in 1972. But maybe we’ve come as far as humanly possible and need a little help as we pull into the last station, the final frontier of efficiency.
Keeping an AI on the (rail)road
Imagine being able to spot every fault over the vast expanses of every railroad line. Then building a large enough data set and analysing it to predict the next fault, while enabling this intelligence without disrupting train schedules.
Could there possibly be a day where we report zero incidents in light- and heavy-rail transport? It’s a question we often mull over at Ignitarium’s R&D lab, ‘The Crucible’ as it is called. A couple of years ago, we sensed the opportunity to put a higher sense in the sky: aerial analytics. To do what we couldn’t before terrestrially, we would now do aerially – cheaper, faster, better.
Imagine being able to spot every fault over the vast expanses of every railroad line. Then building a large enough data set and analysing it to predict the next fault, while enabling this intelligence without disrupting train schedules. And doing it all with limited operator intervention. That’s a tall order. The sheer challenge made it a use case worth exploring. But before we hopped onto this video analytics wagon, which was set to grow at 42 per cent annually by 2022, the question was: we’ll cross the viability barrier, but how do we make it scalable?
Innovation, from the yard to the junction
Iron Man may have Jarvis, but our teams generally have to work with more science than science fiction. Here’s the ‘stark’ reality: hours in the lab are long, and prototype building is an endlessly iterative process of elimination for which one must toil tirelessly. As we tinkered with beta versions in the Ignitarium lab, we tried to ace the first test of any AI solution: running it without human supervision. Aerial analytics obviously meant mounting a camera on a drone or a fixed-wing aircraft, but how would we train it to be highly accurate irrespective of variations in movement?
The first step was to precisely capture the artefacts of interest, compensating for aircraft pitch, roll and yaw. Once that was done, we ensured no operator would be required to control or maneuver the camera system. This was achieved by adding the captain’s wings to Ignitarium’s dynamic camera alignment software. What we needed now was a way to acquire high-quality video such that every rail-track-related object of interest would be perfectly ‘centered’ in every single captured frame. Working with our hardware partners, we found a powerful device up for the mission: an AVerMedia Box PC EX731-AAH2-2AC0, with an NVIDIA Jetson TX2 module inside.
Once the aerial vehicle and gimbal were up in the air, the rail track footage was stored in 4K resolution for granular analysis. Complex image processing algorithms were written and rewritten for image registration, detection, and classification, all working in parallel on TYQ-i, Ignitarium’s defect detection AI platform. At first, the AI was trained to look for obvious faults in the track: cracked or skewed ties and missing spikes or fish plates. Then, as it learned more, it was time to go deeper: see and tag foreign objects and even vegetation of all kinds. After multiple beta builds, it was time to set our AI free and let it fly.
Gathering steam: tunnel vision, sounding the alarm, and more
With our solution put to work for Skycam Aviation, a leading aerial imaging company in the U.S., we felt a sense of pride. And yet, the journey was only beginning. Our team switched tracks to other obstacle detection approaches: more work for us, and more processing for TYQ-i. Think of the combined powers of aerial analytics with cameras mounted on trains, trained to spot disruptions even in the dimmest of conditions: night, rain, snow. Or an AI hammer that analyses sound patterns while testing structural integrity inside tunnels to detect abnormalities. And what if the AI could study the historical footage of the visual landscape around a train’s regular route to identify disparities with the current footage?
Our mission at Ignitarium is to make it possible to implement vision analytics economically, at scale. So, the cost of predictive video analytics isn’t even a consideration when weighing it against human safety.
It’s not a what-if anymore: it’s as real as it is efficient. The mechanism is to install a depth detection camera in front of the vehicle. We then select a section of the track to be recorded, after which hundreds of frames from point A to point B are geo-tagged and stored as ‘golden reference’ saved feeds. Now we’re ready. When the train is in motion, the saved feed is synchronised with the live feed to detect differences. There are many paths to greater accuracy, and we are exploring them all: from comparing only key points in both frames, to using accelerometer or gyroscope based encoded data, to getting wheel rotation information from the train itself. Whatever the path, the objective is to make detection possible at ultra-high train speeds without missing out on any small objects. Sharpening this also requires the right definition of a region of interest (RoI) window.
Our mission at Ignitarium is to make it possible to implement vision analytics economically, at scale. So, the cost of predictive video analytics isn’t even a consideration when weighing it against human safety. That, I believe, would make my 21-year-old self really proud. As a newly minted electronics engineer from the Bangalore University, setting out on my rail travels at the turn of the millennium, I rolled up the window from my side berth in the coach. Brimming with optimism for the future, I gazed ahead at the tracks as the train snaked along a steep curve bend. Little did I know that a couple of decades later, we would have sent a drone up ahead to gaze at it with me.
Related topics
Artificial Intelligence (AI), Big Data, Digitalisation, Drones, Track/Infrastructure Maintenance & Engineering