Computer vision based solution for sign detection
Posted: 6 October 2016 | | No comments yet
Markus Melander, CEO of Vionice Ltd., discusses the opportunities of computer-vision signage which may help increase asset management quality and railway safety at the same time.
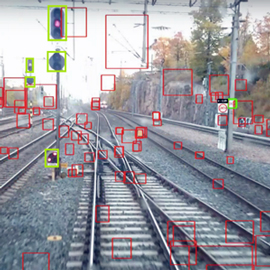
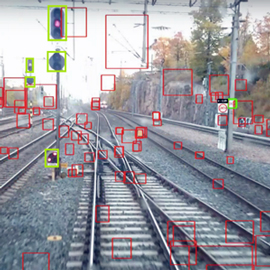
Auditing the Finnish railway network, a total of 12 000 km, took only a few months with the help of mobile phones, computer vision, and a cloud-based situational awareness tool.
In this article we present a solution which reveals interesting possibilities in increasing asset management quality and safety at the same time. To get the best out of the technology, some cross-organisational cooperation is required. The core message of this article is that in some cases, ordinary rolling stock can collect a bulk of the data needed for track asset management and mobile devices are enabling data gathering at a lower cost.
Computer vision has been increasingly used in railroad inspections and maintenance in some application areas such as track integrity inspection and pantograph surveillance. There are also applications for counting and surveillance of rolling stock on non-automatically controlled tracks.
The business case at hand portrays our experience in mobile video production and computer vision detection for the inventory and quality assurance of speed indicating signs. The project took place in November 2015, which is one of the darkest periods of the year in Finland.
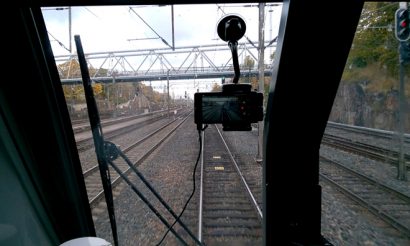
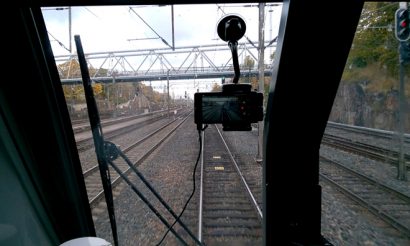
Mobile phone gathering video data. (image: VR Track)
The driver of the train requires constant information of speed limits. The information is indicated via Automated Train Control systems and by speed-indicating signs. The signs act mainly as a backup in order to ensure traffic during anomalies. VR Track designed and planned the renewal of track signage according to the renewed instructions of the Finnish Transportation Agency. The actual removal of some signs took place afterwards.
The solution can be as simple as a mobile phone
New mobile technology enables a lot and provides low cost alternatives. When coming to low cost methods, an interesting detail in the case project was that the track videos were collected with a simple mobile phone application. There were a few advantages obtained from this: 1) The raw video data collection was done in a fraction of time when compared to the use of external equipment, 2) Ordinary passenger trains and cargo trains were used for the raw data collection. Arrangement was cost effective and plenty of time was spared.
The project revealed that smartphone cameras are accurate enough and are able to provide good quality images and videos. The produced material was suitable for further automated computer vision analysis! However, limited lighting conditions brought some challenges for automated data extraction. In addition, a diesel locomotive’s shape blocks a big part of the view. Nevertheless, when the locomotive´s frontal lights are on, the signs were detectable due to the reflective surface of the sign. Diesel locomotives and limited lighting made some videos unusable for later viewing but even with these challenges the computer vision was able to yield the same result: a correctly detected speed sign. This is why some of the produced material is not usable for further track environment viewing but works perfectly for sign detection.
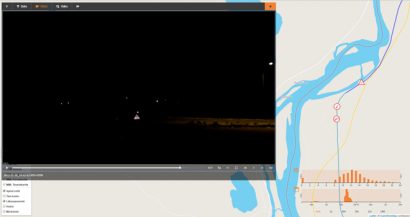
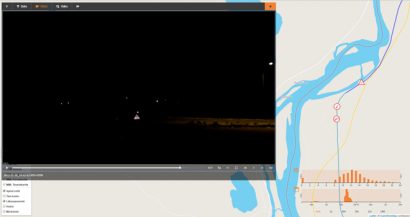
Darkness is not a problem for computer vision. (Image: Vionice)
The main practical advantages using mobile phones were:
- It is very easy to start filming
- Videos can be shot from multiple trains at the same time,
- The mobile phone based video is available on the situational awareness tool’s map interface within a few minutes.
Consequently, this unfolds other interesting possibilities for real-time track-level video usage but is possible only if 4G networks are available along tracks or if the train has a good enough data link onboard.
Computer vision is effective
In general, it is good to be aware that computer vision and machine learning are currently able to detect basically the same issues from the videos as humans. The main difference is that for the machine, the contextual detection is much harder – almost impossible. It means that a computer does not make assumptions or cannot extrapolate information like humans can. Computer vision technology uses data sets for learning. Without any pre-information the computer vision is not able to classify objects.
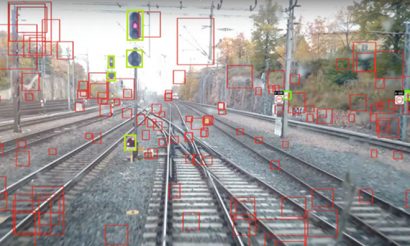
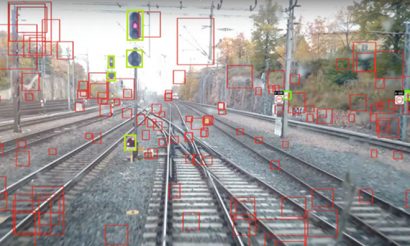
Computer vision in action. (Image: Vionice)
As in the presented project, computer vision is mainly used for visual inspections during labour centric processes. The added value from using automation comes from multiple factors, in short:
- Computer vision does only what it has been taught to do. It does not guess or make assumptions.
- All the detections can be valued under a certain confidence level. If the accuracy of detection is not confirmed, the software will propose a human operator to verify the detection.
- Possible mistakes can be tracked and can be fixed. Modern systems are mostly still learning so they become more accurate when facing similar circumstances or situations.
- Computer vision system is tireless. It can do the extraction work without getting tired or distracted.
In this project the signs were extracted from the videos and were localised with photogrammetry. The positioning is accurate enough in some of the cases but in future applications the image based 3D point cloud can be used to enhance the accuracy in positioning.
Conclusions and lessons learned
Up-to-date visual material, whether it is a HD video or high resolution images, is highly valued by multiple stakeholders conducting some kind of work on the tracks. Images can be used for browsing and checking locations along the tracks. There is a huge advantage if the images are up-to-date. This concept also provides the possibility to produce and browse seasonal images that might be valuable in some cases.
There is clearly a need for better situational awareness of the railway infrastructure. It comes down to how often we can update our images from the track in a cost-effective way. Easy use of video information has already decreased the need to physically go-and-inspect. The next phase is to replace other tedious, labour centric, recurring tasks with computer vision analysis based solutions.