DB’s advances in Condition-Based Maintenance and failure forecasting for rolling stock
Posted: 16 March 2017 | Burkhard Schulte-Werning, Heiner Mösken (DB Systemtechnik GmbH), Patrick Linke (DB Systemtechnik GmbH) | No comments yet
Today’s maintenance systems for rail vehicles and infrastructure are characterised by a combination of scheduled maintenance and unscheduled repair. Preventive maintenance entails inspection based on pre-defined intervals with replacement or overhaul of components being carried out before failure occurs, and corrective maintenance with repair or replacement happens in the event of actual failure. In this article, Burkhard Schulte-Werning, Heiner Mösken and Patrick Linke at DB Systemtechnik GmbH highlight the benefits of utilising the more evolved alternative of condition-based maintenance and share advances that have been made in this technology at DB.
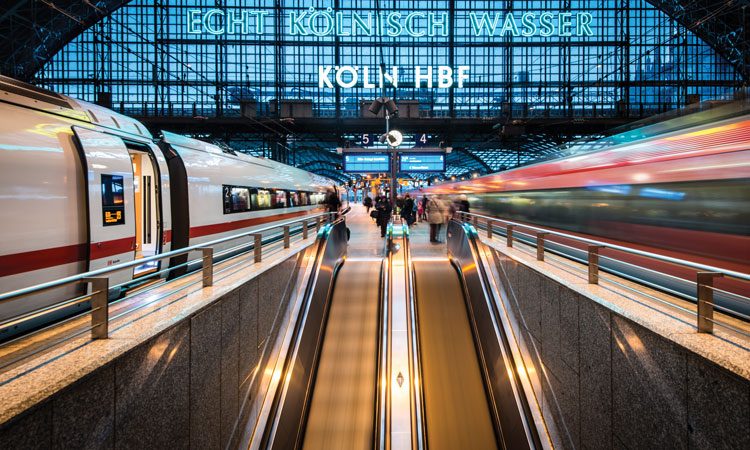
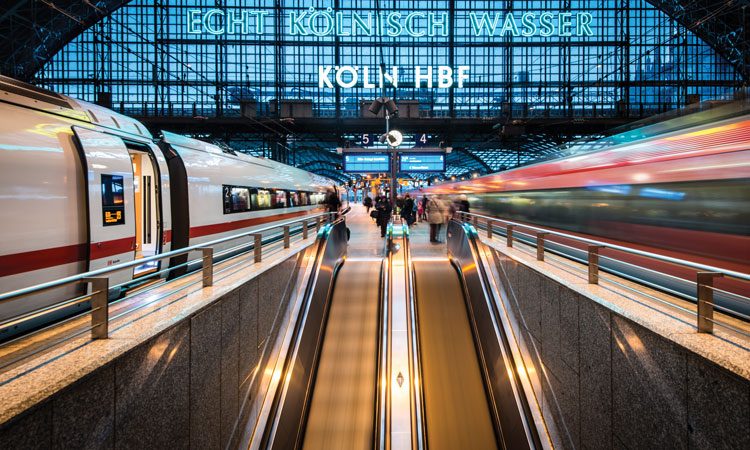
Further potentials for higher availability and reliability of rolling stock, as well as lower costs of maintenance, can be realised by migrating towards Condition-Based Maintenance (CBM) with the aid of monitoring and forecasting based on existing operational and condition data (see Figure 1). As a result, greater use can be made of wear reserves and costs can be cut. Reducing unscheduled failures increases the reliability of assets and, thus, their availability to perform
the transportation task.
The aim of CBM is to plan and implement maintenance measures that are deemed necessary due to the condition of rolling stock in a manner that is optimum in terms of time, quality and cost aspects. Using modern measuring instruments, the condition of rolling stock can be recorded, described and evaluated permanently. Thanks to this information, root causes of possible disruptions and damage can be detected in advance and eliminated ahead of time.
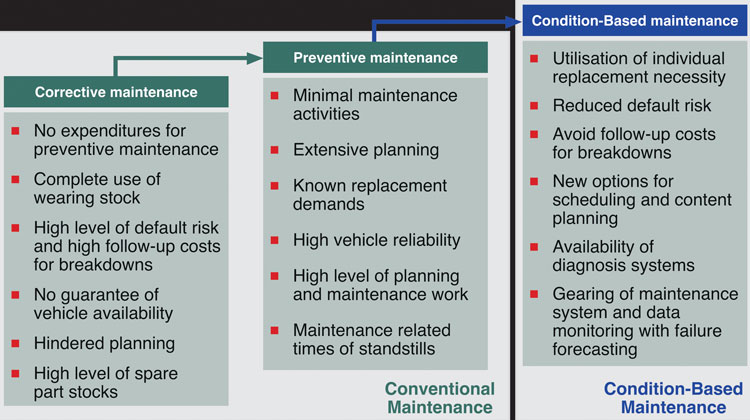
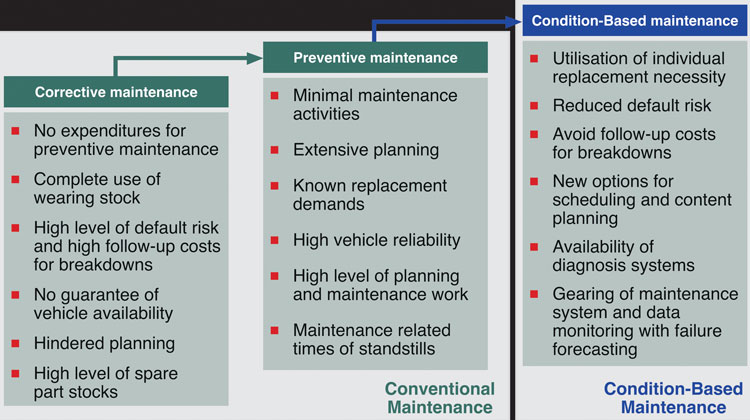
Figure 1: Automated condition evaluations with forecasting based on existing data from diagnostic systems is the next step in the enhancement process of maintenance systems
CBM application areas in the rail sector
Four application areas of CBM are specific to railway and rail infra structure companies for the purpose of monitoring assets1:
- Vehicle monitors itself
- Vehicle monitors the infrastructure
- Infrastructure monitors itself
- Infrastructure monitors vehicles.
For the ‘vehicle monitors itself’ application area, process values from the individual subsystems required for open and closed-loop control in addition to those coming directly from existing sensors can be used. Recently installed rolling stock already possesses on-board diagnostic systems that regularly generate operational data. This enables maintenance costs to be reduced by means of CBM because vehicle components’ wear reserves can be fully exploited and forecast maintenance measures can be scheduled by coordinating with engineering at the depot.
‘Vehicle monitors itself’ application area
In order to answer generic questions of CBM on rolling stock equally affecting all carriers within Deutsche Bahn (DB), DB Systemtechnik was commissioned to carry out a project (see Figure 2) to gather experience of CBM; specifically the following:
- Selection and example use of on-board diagnostic systems’operational and condition data, that is already in place in modern rail vehicles with the capability to access the data
- Analysis of this data to detect hidden patterns to enable the enhancement of maintenance specifications
- Translation of findings into recommended actions for maintenance
- Clarification across business units regarding fundamental questions of data provision, analysis methods and utilisation of results for optimising maintenance.
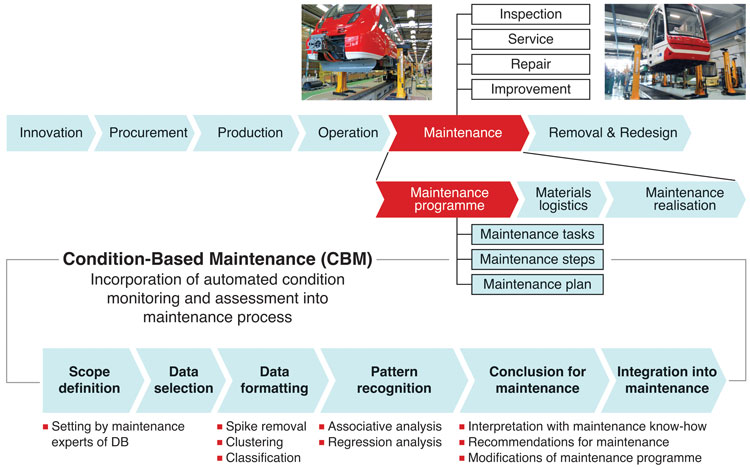
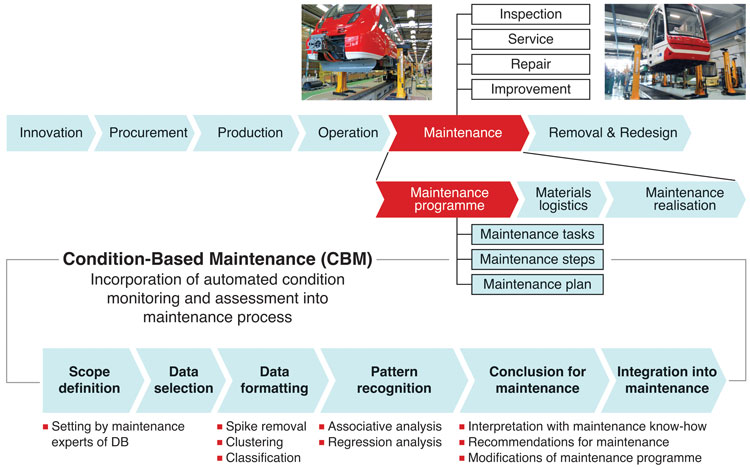
Figure 2: Data gathering circuit in operation, data analysis and pattern recognition and also derivation of conclusions for maintenance and its implementation
Modern rail vehicles already possess on-board diagnostic systems that continuously generate operational data. Process values from the train communication network (TCN, with vehicle bus MVB and train bus WTB), which are required for open and closed-loop control and that come from existing sensors, will be used for diagnosis in the individual subsystems. If the data is accessed and analysed regularly, this monitoring of vehicle systems and components will enable CBM to be carried out.
The traction control unit (TCU) is the central processing unit for the converters and essentially breaks down the traction motors’ output. If the TCU fails, tractive effort is reduced. Depending on the route and the weight of the train, continued travel is restricted and the train must be taken to a maintenance depot. This results in substantial delays and follow-up costs.
The door control comprises the systems of a rail vehicle that control the unlocking, opening, closing and locking of doors. To this end, modern trains have a microcontroller installed in each vehicle as a so-called door computer. The technology-based passenger handling process currently used in Germany operates as a door-closing process, in which doors automatically monitor correct opening and closing and detect persons or items trapped in the doors. Therefore, disruptions in the area of the doors have a direct impact on boarding and alighting; passenger throughput; and thus the punctuality promised by the rail company and the overall transport service it provides.
By means of statistical pattern recognition, regular patterns, repetitions, similarities, interconnections and trends etc. detected in sensor and event data are identified as correlations and can be used to forecast faults. In this way, such pattern recognition processes supplement the classical optimisation strategies for maintenance that rail and rail infrastructure companies currently use to enhance their maintenance plan on the basis of their experience of operation
and maintenance.
In the aforementioned project, all event data, such as condition and fault messages from Class 442 regional electrical multiple units (Bombardier Talent 2) and Class 145 locomotives (Bombardier TRAXX electric locomotives), were analysed by means of statistical processes. On the basis of this data, conclusions are reached about the current condition of the vehicle and its components, and a forecast of future system health is also made; i.e. if the current condition
indicates that a particular disruption is about to occur. A retrospective anomaly evaluation (retrospective evaluation of irregularities and control deviations) and a prospective disruption forecast (time forecast of future defects) are performed on the basis of a set of event data.
Beginning with a reasonable volume of (test) data from the vehicles considered, modern statistical algorithms are applied2 to orientate the analysis processes to them and to train these on them. This training experience is then used to perform the disruption process on the basis of regularly arriving condition data and to validate the process. Thus, in total a circuit of data gathering and analysis, trend recognition and implementation in maintenance is set in motion that incrementally enhances the precision of the process. Anomalies should be flagged up as early as possible to boost the detection rate compared with that of conventional evaluations and to avoid false alarms (see Figure 3).
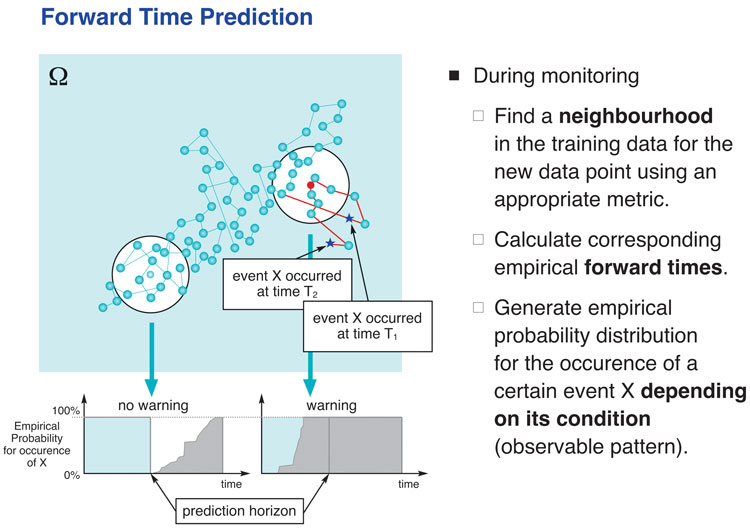
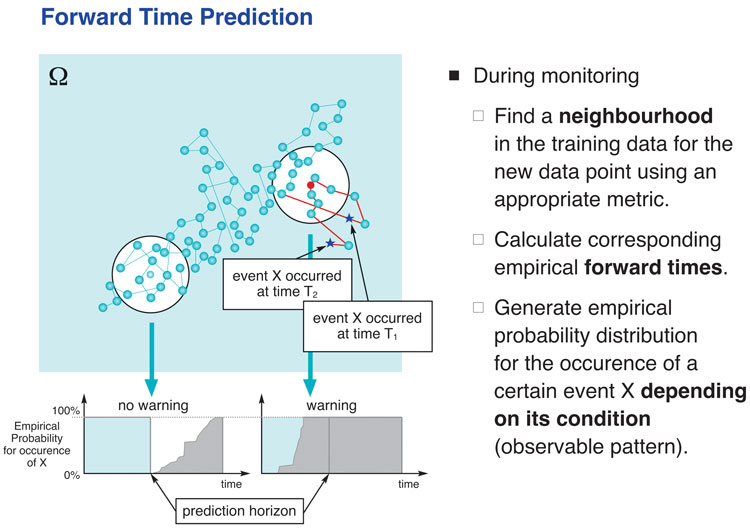
Figure 3: Forecasting disruptions with empirical probability distribution based on observed time pattern
The analyses presented here refer to data that was recorded over the course of several months in order to be able to begin the process of modelling. In the case of events that occur more frequently, shorter recording time periods suffice to provide an adequate basis of disruption examples. For more seldom events, recordings must take place over longer periods of time to enable evaluation of a sufficient number of disruptions. The amount of data required at the start can therefore not be defined a priority, but is determined heuristically for the forecast model. All arising forecast models can be adapted and improved on a regular basis by means of subsequent continued data gathering.
Events such as diagnostic codes 0642 ‘No ready message from TCU 1’ of Class 145 and ZNAA ‘Doors’ of Class 442 can be forecast with ease. The process is based on the concepts of cross-correlation and similarity sampling and is implemented efficiently by using fast Fourier transformation. The programme, known as EventSimulator, is ready-for-use and can be applied routinely to event data. At present, PDF reports containing the sections Detection, Retrospective and Prospective Forecast are generated and sent. Automatic learning based on sensor data is similarly possible to learning based on event data. The process can be advanced in two ways: by determining optimum distances to define the neighbourhood of a system condition, which leads to steeper and more distinct condition distributions; and use of whole fleets for training, which leads to smoother condition distributions. Consequently, more precise probability statements on disruptions and their forecasts are thus possible overall.
Considered approximately, forecasts of abnormal conditions are made with an over 90% justified warning rate and fewer than 10% overlooked events (see Figure 4). The mean forecast advance warning time is approximately 100 hours – a lead time that is adequate to allow the maintenance depot to schedule work orders. The detected data patterns can be used to enhance maintenance specifications and recommended actions when carrying out maintenance.
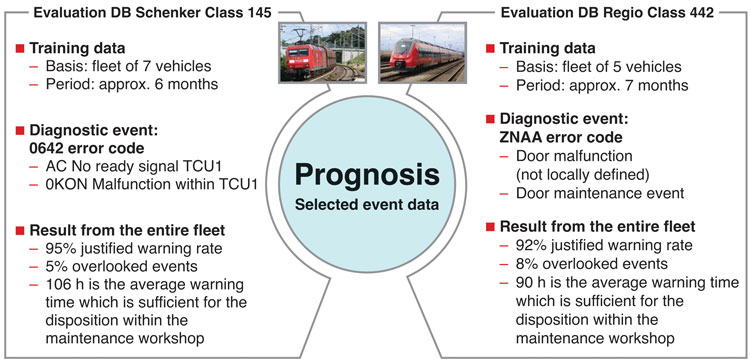
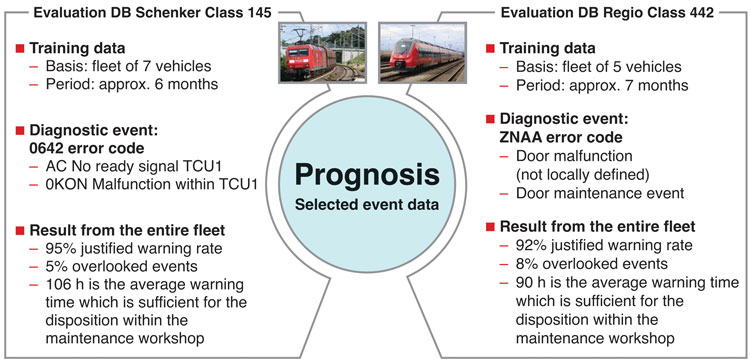
Figure 4: Results of data analysis on two selected event codes of the Class 145 and the Class 44
The process learns from historical data and disruptions that have occurred. It is important that all incidents are registered and recorded correctly at the depot. As such, during training of the models, steps are taken to ensure that only components that are actually defective are trained as such, to ensure the learning result is not detrimentally influenced.
Integration in maintenance
When it comes to reducing maintenance costs and boosting availability and reliability of rolling stock, the advantages of CBM can be realised whenever the data gathering and condition monitoring process is integrated into the operator’s maintenance plans. These must be defined by the rail company on the basis of the manufacturer’s maintenance plan in accordance with its operational requirements and experience with operation. Of course, the requirements settled in prEN 17023 must also be fulfilled3. This opens up new options for making it possible to plan in terms of time and content. Improved exploitation of the useful life of components and their wear reserves can give rise to an automated new concentration of measures in flexible maintenance levels.
References:
- Schulte-Werning, B.; Mösken, H.; Linke, P.; Hoksch, V.; Uebel, L.; Wolter, K.-U.: On current Developments in Condition-Based Maintenance at DB. Proceedings of 11th World Congress on Railway Research 2016, Paper 450, 29 May-2 June 2016, Milano, Italy
- Mergenthaler, W.; Jaroszewski, D.; Feller, S.; Laumann, L.: A joint renewal process used to model event based data. Decision Analytics (2016) 3:2, DOI 10.1186/s40165-016-0019-9, SpringerOpen Journal
- Technical Committee CEN/TC 256 ‘Railway applications’: prEN 17023:2016: Railway applications – Rolling stock maintenance – Creation and modification of maintenance plan, 2016.
the field of rail freight services, maintenance technology and gear production. He has held responsible positions in development, assembly, maintenance and IT-Systems. Today, Patrick is a member of the DB Systemtechnik expert organisation and TSI representative for Maintenance Technology. As Senior Specialist in Maintenance he has gained special experience in project management for CBM.
Issue
Related topics
Big Data, Digitalisation, Internet of Things (IoT), Rolling Stock Maintenance, Rolling Stock Orders/Developments