The role of digitalisation and innovation in the Rail Baltica project
Posted: 8 September 2022 | Andy Billington | No comments yet
Andy Billington, Innovation and Sustainability Expert at Rail Baltica, explores what options there are to deploy sensor technology along the project’s infrastructure and what potential there is for building a ‘digital native’ railway.
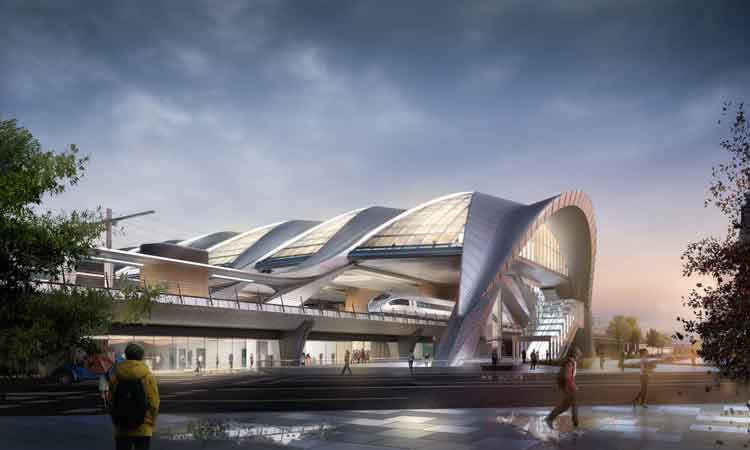
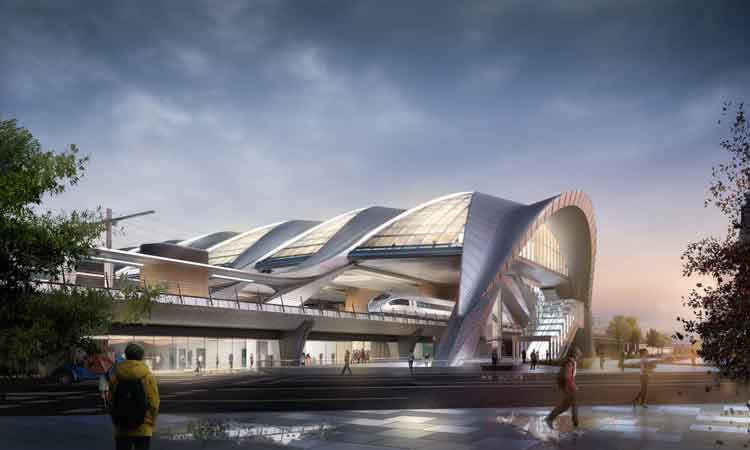
Riga Central Station, aerial view south. Credit: railbaltica.org
Rail Baltica is the largest railway infrastructure project in the history of the Baltic States, offering unique opportunities through linking Estonia, Latvia and Lithuania with a standard gauge railway connecting to Poland and beyond, the new mixed-use line, bridging the missing link of the North Sea‑Baltic core network corridor, will support a range of passenger and freight services – connecting cities, towns, ports, and airports, and serving both to people and businesses. This is a greenfield project; the infrastructure and all the systems are being developed using modern approaches, with extensive use of BIM and other tools, and with both digitalisation and sustainability as key factors.
Digital infrastructure options include rural/ regional connectivity (such as 5G and broadband), backbone networks, services at stations, and connectivity for socio-economic drivers, while energy synergies will cover grid resilience, standby power, options at regional stations (for example, for electric vehicle charging), renewable sources and other emerging opportunities.
Potential sensors
The systems design for Rail Baltica is at a relatively early stage and a lot of decisions lie ahead – as do a lot of opportunities.
While the systems design for Rail Baltica has started, key decisions about the types of sensors to be implemented have yet to be made. The range is clearly wide, from railway operations to asset management and monitoring: some are safety-critical, others not; some have very strict cyber-security requirements; power and communications requirements vary, and there are architectural considerations such as designing systems to provide an integrated view with all available information from the railway, regardless of source.
Options which have been studied include distributed acoustic sensing (DAS), vehicle identification systems, weighing-in-motion, and a range of smaller sensors: each of these have different characteristics in terms of data volume and velocity, with some providing real-time information and others reporting only on change. DAS technology provides a way to monitor both the structural health of the railway infrastructure and some properties of the vehicles (for example, wheel flats): a train running over a given line section will cause vibrations which are detected by the impact those vibrations have on a signal in the fibre. Information which can be made available includes location and direction of traffic, speeds, track and track bed conditions, fastener issues, flashover events, and certain wheel defects. Such systems provide the capability to monitor 50km (or more) of track from a single monitoring location, with no requirement for wayside equipment other than optical fibre: it is also possible to monitor that distance in either direction, reducing the number of equipment locations needed. The data volumes generated are significant, so processing nodes are often located at the monitoring locations with the potential for transferring specific events in real time without having to move bulk data as it is captured. However, this bulk data also has the potential to provide a useful input for ‘big data’ analysis and machine learning, especially if DAS data can be correlated with other sources.
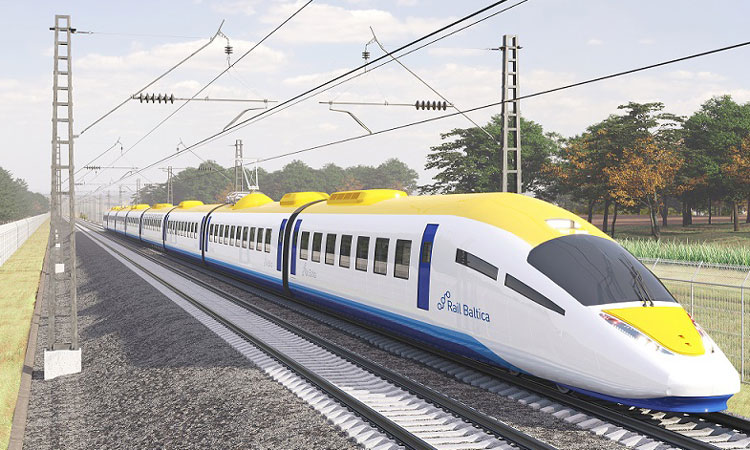
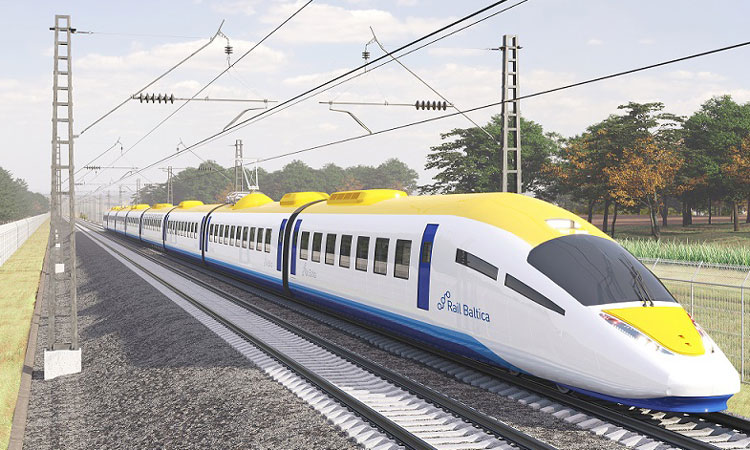
Rail Baltica train concept. Credit: railbaltica.org
For vehicle identification, there are several options, but one of particular interest is the use of RFID at selected locations: while a ‘video gate’ can capture a great deal of information (for example, images of any hazard warnings on freight wagons), the size and complexity of such solutions limits their feasibility in open line sections. In contrast, RFID readers can use the approaches described in EN17230 (and elsewhere) and can provide vehicle identification for other sensors by positioning readers alongside other monitoring systems. Provided the identifiers used for each vehicle are both unique and consistent (for example, the same type of vehicle identifier is used at a video gate and a wayside reader), then the information from multiple sensor types monitoring multiple vehicles can be correlated across the entire line. In addition, a similar approach to standardised identifiers can be used to assist with asset management and maintenance, both for operational purposes and for supply chain management.
There are also Internet of Things (IoT) sensors, with a variety of solutions that can be used for monitoring things such as ambient environment at stations…
Weighing-in-motion (WIM) is also typically done at specific checkpoints at low speeds and could possibly be co-located with video gate systems: however, with newer, more capable systems, monitoring at higher speeds has become possible (for example, measurement of regional passenger trains at line speed). This data could also be correlated with vehicle identification to provide specific information regarding weight, vehicle types, uneven load or overload, warn of increased derailment risk, and some types of wheel damage. Information can also be compared across measurement sites to identify any changes in real time.
There are also Internet of Things (IoT) sensors, with a variety of solutions that can be used for monitoring things such as ambient environment at stations (including noise levels, air quality, and more), and some emerging solutions for rail monitoring using energy harvesting. These sensors typically connect over lower power wide area networks, whether that’s NB-IoT, LTE-M, or other options such as LoRa: with the emergence of 5G networks, other options and more services also become available. One key element of the overall architecture is to ensure that sensor data can be integrated, no matter what the connection, or whether real-time, near real-time, or bulk data.
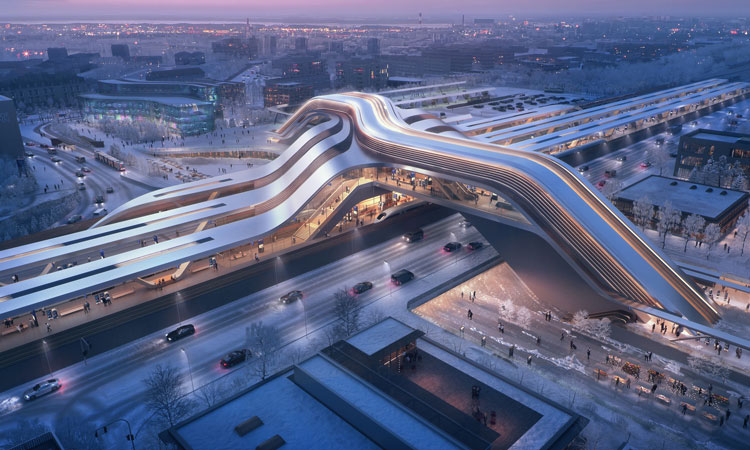
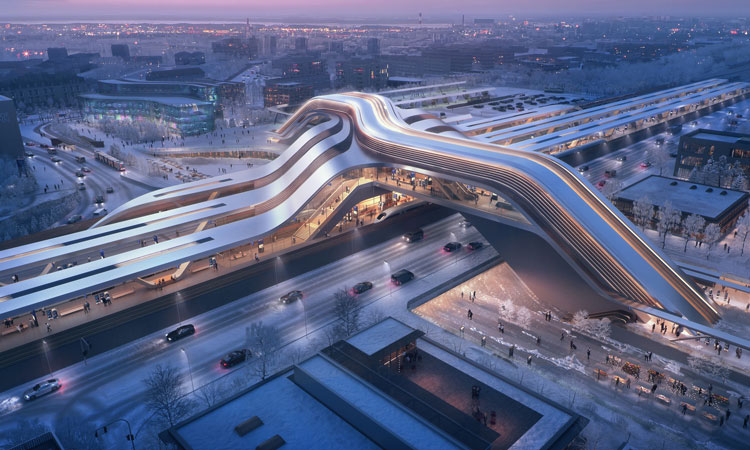
Ulemiste Station, Tallinn. Credit: railbaltica.org
Data integration and the importance of standards
One key aspect is data integration: there are some standards, but bespoke and proprietary solutions are also common in rail. However, it is important to note that the overall direction of the European rail sector should be towards modular systems and common standards: several projects managed by Shift2Rail demonstrated the use of ‘off the shelf’ software and solutions, and Europe’s Rail Joint Undertaking (the successor organisation to Shift2Rail) is building on the outputs of its predecessor. This overall direction has several advantages, including reduced vendor risk, increased flexibility, and options for better lifecycle management of systems, so that for example, if one component needs an upgrade, it is not necessary to upgrade an entire system.
Common integration tools can be used for non‑critical data, and using an appropriate security architecture, silos can be avoided: a very rich set of real-time and bulk data can be made available for monitoring, managing, and maintaining the infrastructure.
Analytics and machine learning
The value in collecting and correlating sensor data is clearly linked to the ways in which the derived information can be used. There are many different potential applications, and with an appropriate architecture, more can be added as they emerge. Machine learning algorithms can support anomaly and outlier detection, clustering, classification, and so support predictive maintenance: with standardised identifiers throughout, data from different systems can be correlated to support broader application of these techniques.
One key element of the overall architecture is to ensure that sensor data can be integrated, no matter what the connection, or whether real‑time, near real-time, or bulk data.
There are decisions to take about the longer‑term data management, with options such as an enterprise data warehouse, a centralised data lake, Lambda (or Kappa) architectures, or the newer data mesh approach. For a distributed environment such as a railway, a single enterprise data warehouse or centralised data lake may have significant drawbacks, especially as data sources and data consumers are likely to be in many different locations, not always at that ‘hub’, but also because of the sheer volume of data that could be generated, and the difficulties of managing both access and data quality in a single centralised pool. Remote sensor processing (for example, DAS) can lead to events being sent to a core system, and this is likely to be as a stream (rather than in batches), so architectures which can manage streaming sources natively (Lambda, Kappa, mesh) are better suited: some other sensors may only send updates on change (for example this is a feature of many industrial monitoring solutions) or on an ‘out of normal range’ value, and again this is likely to be event data, rather than a batch. The Lambda approach can manage both streams and batches, with the Kappa architecture being a more specialised version aimed at streams: both have advantages and drawbacks, but are more suited to a distributed environment than the previous centralised approaches. The fourth main approach is the newer Data Mesh paradigm, which includes support for full decentralisation, for data ownership and governance to remain with the team responsible for generating the data (not being transferred to a central team which may not have the same ‘domain knowledge’ as the specialists in their field) and treats data as a product. In a complex environment such as a multinational railway, this approach can allow for the separation of data ownership while still allowing for the advantages of scale and the use of common tools, allowing all parties to share data safely and securely and benefit from each others’ experience, which could be especially useful for predictive maintenance.
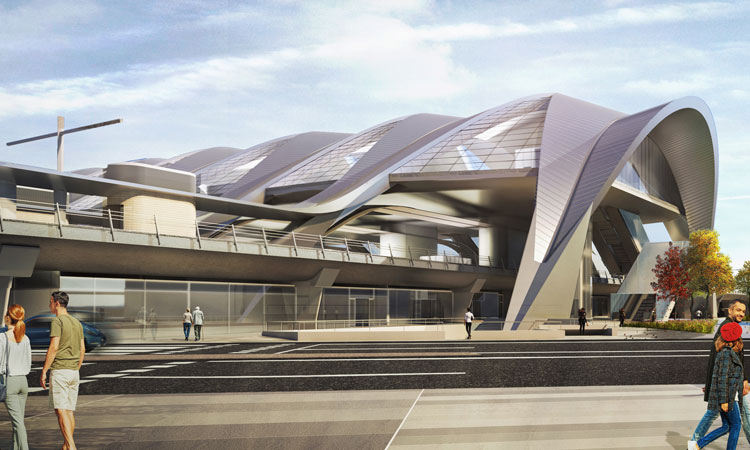
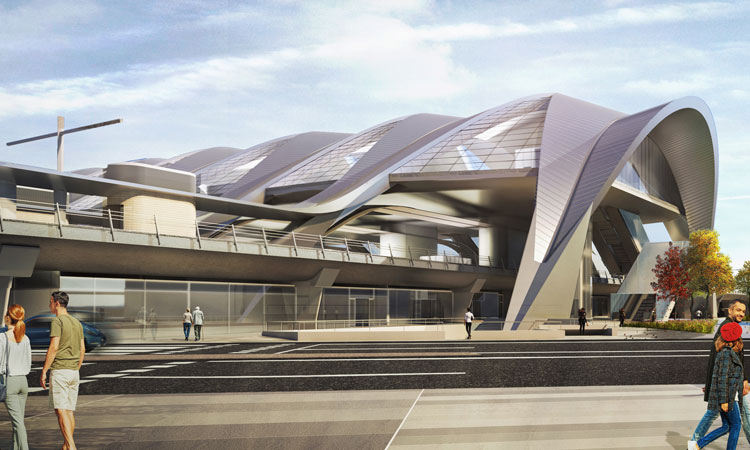
Riga Central Station from Gogola street. Credit: railbaltica.org
Of course, there is also the cloud, or ‘on‑premises’, element to consider: cloud lock-in is a very real concern, with more and more large enterprises looking at hybrid and/or multi-cloud approaches. The mesh paradigm also allows for these configurations, and for a solution which uses a distributed ‘on-prem’ cloud for operational data, with less sensitive systems potentially going to government or public cloud services. Such an internal cloud could be deployed with relative ease using exactly the same approach to hardware and connectivity as the larger ‘hyperscalers’ such as Azure, AWS and others, aiming to minimise energy use and maximise efficiency. In such an environment, systems as well as machine learning algorithms can consume the data products from various sources, without an unnecessary (and typically expensive) centralised data warehouse: if the data ‘products’ are self-describing and discoverable, then a domain specialist in one field who wishes to combine their data with that from another field could turn to a ‘self-service’ platform in that internal cloud.
The potential for building a ‘digital native’ railway is significant
As aforementioned, the systems design for Rail Baltica is at a relatively early stage and a lot of decisions lie ahead – as do a lot of opportunities. The potential for building a ‘digital native’ railway is significant: it should not be lost or restricted by an adherence to older approaches such as enterprise service buses or a single centralised data warehouse. Unlike many ICT systems, railways do not have a two-to-three-year lifecycle but more typically one of 20-30 years: modular systems should be designed that allow for innovation over time. As new classes or types of sensors are developed or as new machine learning algorithms become available, there is clear scope for their outputs being a self-describing ‘product’. Such modular, standards-based, and open approaches could support the operational railway for years, if not decades, to come.
Issue
Related topics
Big Data, Building Information Modelling (BIM), Cyber-Security, Digitalisation, Infrastructure Developments, Internet of Things (IoT), Signalling, Control & Communications, Sustainability/Decarbonisation, Technology & Software