swissTAMP – big data in proactive track asset management
Posted: 23 November 2016 | | No comments yet
For Swiss Federal Railways (SBB), swissTAMP is a key component in building a proactive asset management system vital for the efficient and effective planning of systematic track maintenance.
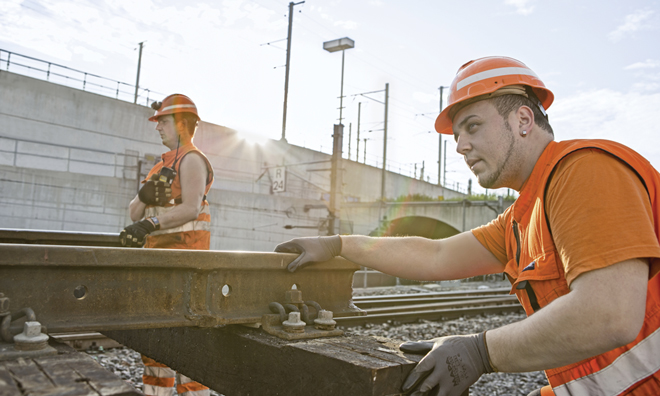
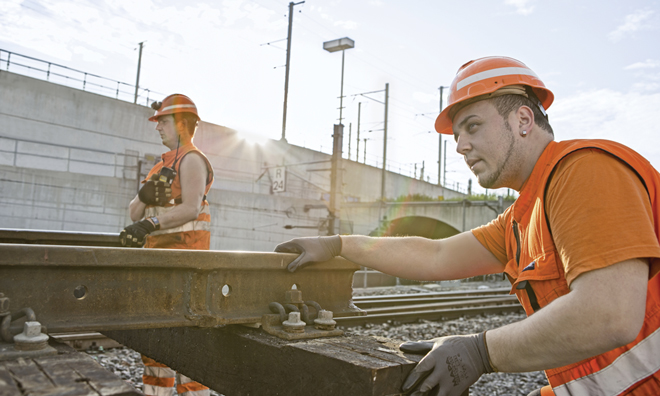
SBB can look back at decades of successfully developing the capacity and quality of its transport services. By selectively expanding its infrastructure, SBB has introduced additional connections and services, while improved timetables and more powerful vehicles have resulted in shorter journey times and punctuality has been steadily improved with low intervention times. An international comparison shows that SBB has been operating the network with the densest volume of mixed traffic in the world for years.
Track maintenance backlog
Over the past 30 years the load on the SBB network has increased by approximately 70%. According to long-term planning of services and resources, the load will again increase by around 70% by the year 2040. Figure 3 shows that, despite the increase in load, track maintenance has in fact been reduced over the same 30-year period, leading to a considerable increase in premature rail replacement and a noticeable reduction in the economic lifetime of tracks and points. When combined with renewal volumes, which have also been too low, the result is an accumulated track-related backlog to the tune of some CHF 1660 million1. The track has to be kept operational and available through increased expenditure including emergency measures required at short notice. During these years, SBB has switched quietly from a preventive to a corrective maintenance regime. The proportion of ‘uneconomical’ maintenance has risen and drawn closer to the maintenance volumes that can be planned and made available for preventive maintenance. In order to put a stop to this downward spiral and be able to cope with the rising demands, SBB needs to switch back from reactive to proactive maintenance2.
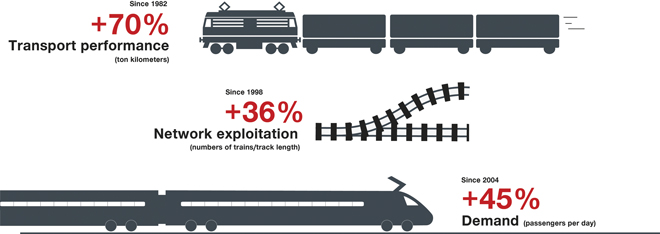
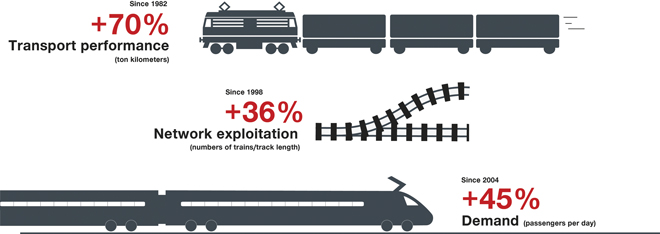
Figure 1: Increasing provision of services on the SBB network
As a first step in the transition back to proactive track asset management, SBB put in place the necessary transparency and knowledge about asset behaviour throughout the network. This was carried out by developing the SBB Standard Elements3 and implementing them in a network-wide strategic forecast model4. Based on this, the asset managers have developed an investment and maintenance strategy that utilises life-cycle cost calculation in order to determine the strategic requirements in terms of the track volume as well as funding needed for the SBB track network. This convinced the supervisory authorities to gradually ramp-up the maintenance volumes.
As a first step in the transition back to proactive track asset management, SBB put in place the necessary transparency and knowledge about asset behaviour throughout the network. This was carried out by developing the SBB Standard Elements3 and implementing them in a network-wide strategic forecast model4. Based on this, the asset managers have developed an investment and maintenance strategy that utilises life-cycle cost calculation in order to determine the strategic requirements in terms of the track volume as well as funding needed for the SBB track network. This convinced the supervisory authorities to gradually ramp-up the maintenance volumes.
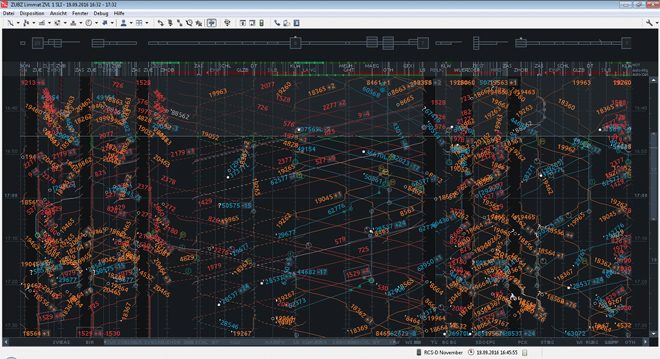
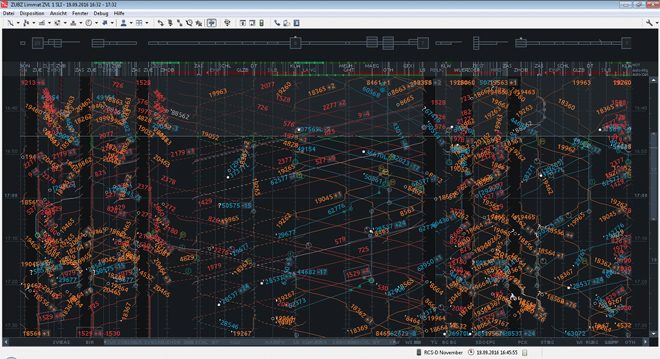
Figure 2: Real-time depiction of rail operations between Zurich and Killwangen-Spreitenbach between 4.30p.m and 5.30p.m.
The grinding volumes were increased by a factor of five while the tamping volumes were doubled. The track renewal volume was also increased by 15%.
The current strategic forecast model is based primarily on the average useful life that can be achieved. This is mainly influenced by the type of superstructure, train paths, load and substructure. It is geared towards determining the strategic volumes for the whole of Switzerland. However, in order to be able to plan measures for particular objects, an asset-specific forecast is required, which also takes account of status data that has been collected and scrutinised on site. The ability to produce a forecast for a precise location, i.e. the method for determining degradation behaviour in relation to the track geometry quality by superimposing measurement data, is now an absolutely fundamental part of modern asset management. The theoretical approaches have been expanded and refined through an array of scientific input5-8. Alongside scientific developments, the method has also been used by other infrastructure managers. Nowadays, the method is implemented in standard software and is regarded as state-of-the-art.
swissTAMP: tool kit for predictive maintenance swissTAMP project
The aim of the swissTAMP project, which was launched by SBB in 2010, was to create a central tool for proactive maintenance. swissTAMP is made up of two components: track analysis and maintenance planning. The track analysis component involves merging a range of measurement and diagnostic data to produce a compre hensive status profile and calculating the period of time remaining until the next measure is required. The maintenance planning component links the track analysis outputs with life-cycle cost considerations and works out optimal proposed measures, complete with financial estimates and possible alternatives.
Data platform for the integration of IT subsystems
Overall, swissTAMP integrates specific specialist knowledge and more than 20 sub – systems of the SBB IT network. The data platform, which was implemented at SBB in 2015, provides the foundation for this. It is largely based on Hadoop9 – a framework for intensive calculation processes involving large and varied volumes of data (big data).
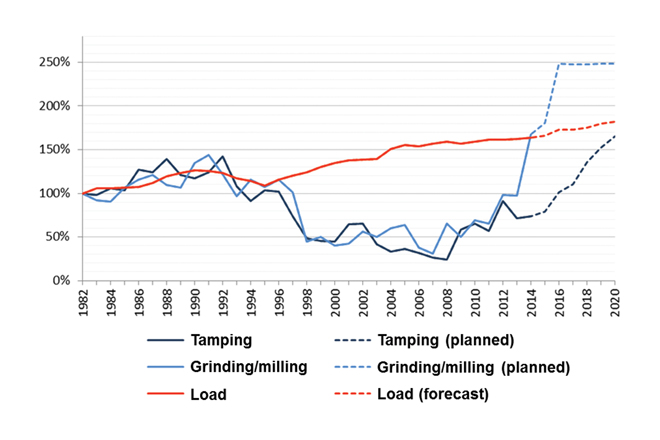
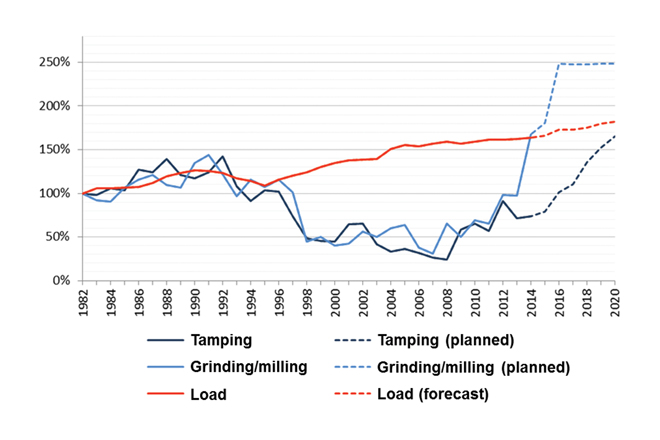
Figure 3: Indexed maintenance volumes between 1982 and 2020 (forecast)
All of the data needed for track analysis is collated and hosted on this data platform. In a subsequent step, the algorithms developed by swissTAMP in the SBB data laboratory to prepare and refine the available data are implemented on the data platform (e.g. localisation and verification). Finally, the functional relationships developed in the business are also computationally implemented on the data platform in order to generate the information that is relevant to the business from the mass of data and make this available to the user on the user interface specially adapted to their needs.
SBB data laboratory: continuous development through specialist knowledge
The track data laboratory serves as the link between data technology administration and operational application. It creates information out of data and generates knowledge. Findings from research and functional knowledge are linked to productive real data and the actual behaviour of assets, driving digitalisation.
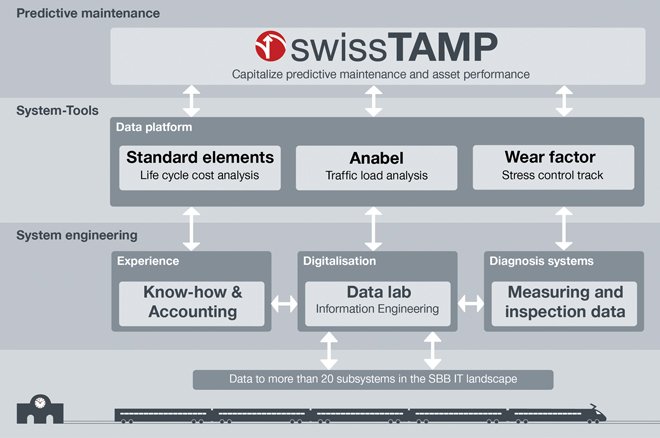
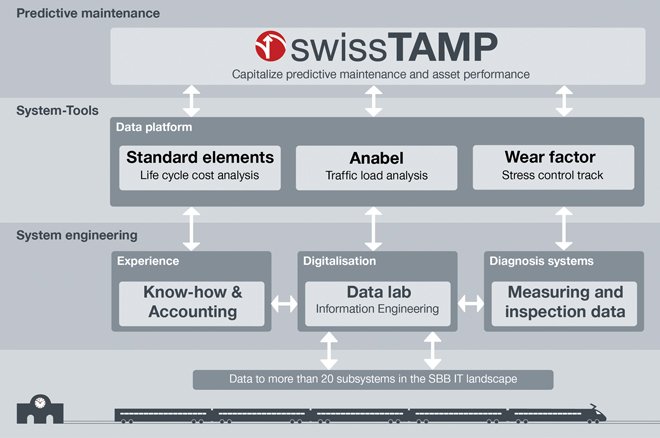
Figure 4: The embedding of swissTAMP in the SBB IT network
An interdisciplinary agile team made up of experts and IT specialists work on developing modules. As shown in Figure 5, these modules are implemented on a data platform like building blocks. By developing new modules a system house is created that allows knowledge about the track system in conjunction with the vehicle-track interaction to be generated, thereby enabling SBB to understand and anticipate the causes of faults, risks and economic trends.
Integration of system tools for proactive asset management
swissTAMP sets the preliminary conclusion in systematically building the toolsets for a proactive asset management. In the process of building these tools data, information and knowledge was developed to make the fundamental change in the maintenance philosophy.
The SBB Standard Elements records SBB specialist knowledge about life-cycles and necessary maintenance measures and matches these with scientific findings3. This tool is used to create standardised nationwide investment and maintenance strategies based on life-cycle considerations. SBB Standard Elements form the basis for transparent and reliable planning of maintenance volumes.
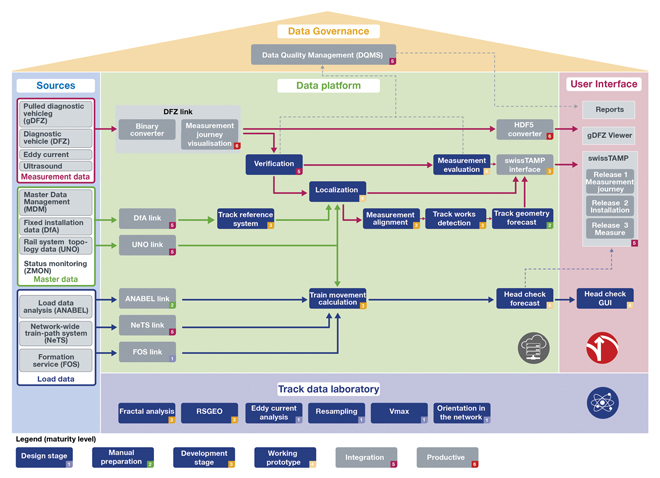
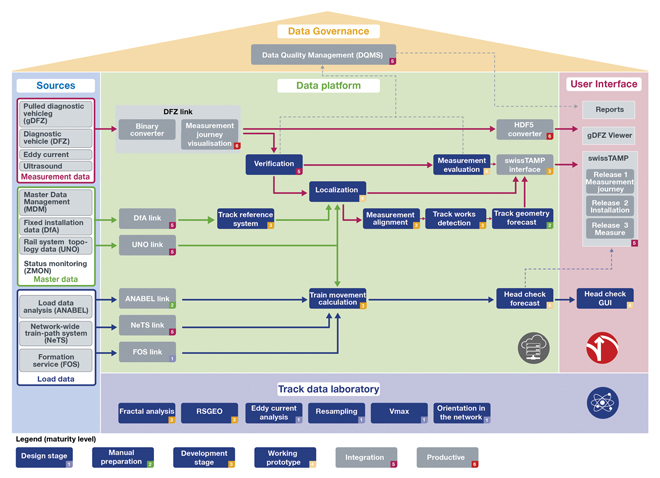
Figure 5: SBB data house with source systems, data platform, user interface and track data laboratory. As per September 2016
The SBB rail control system (RCS) monitors all rail traffic on the SBB track network, thus allowing load data to be collected. ANABEL (analysis and evaluation of load data) is an interface to RCS that enables transparent and traceable monitoring of stress on assets at an unprecedented level of detail. The movements of each train can be recorded in detail. Knowledge about a vehicle’s interaction parameters, including the wheel/track forces, can be assigned to asset components that are travelled on, thus forming the basis for develop ments in track behaviour and calculating wear. This means that wear forecasting and the ability to plan measures for specific tracks are now within reach10.
The ‘track wear factor’ is based on SBB’s track wear model which allows individual vehicles to be classified and their effect or impact on the track to be calculated accurately. The current fixed calculation of overall gross tonnes is thus being expanded to include vehicle-specific calculation of train path prices in accordance with the costs-by-cause principle11.
In the medium- to long-term, the track wear factor will provide an incentive for using the track more carefully and developing vehicles that are more track-friendly.
Practical example: head check forecast
The head check forecast demonstrates the potential that lies in the interaction between the data laboratory, data platform and swissTAMP for the targeted analysis of data that is already available.
Head checks are a typical form of damage which appears on the surface of the rails and is caused by high levels of stress. Head checks are more common in locations where there are particularly high levels of acceleration or braking, or where major traction forces need to be applied.
With its traffic management system, known as the rail control system (RCS), and ANABEL, SBB has systems at its disposal that access train movement data. Building on this data, speed profiles actually travelled are reconstructed and verified in the data laboratory on the basis of block occupancy times, train formations (FOS) and master data (UNO and RCS).
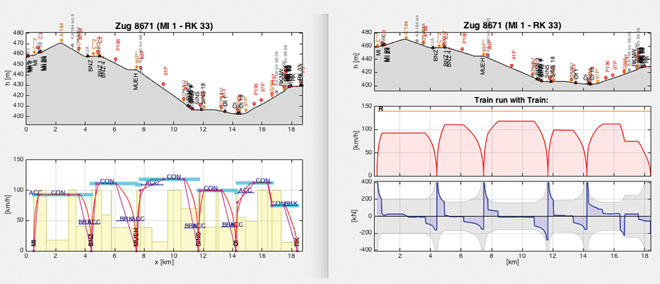
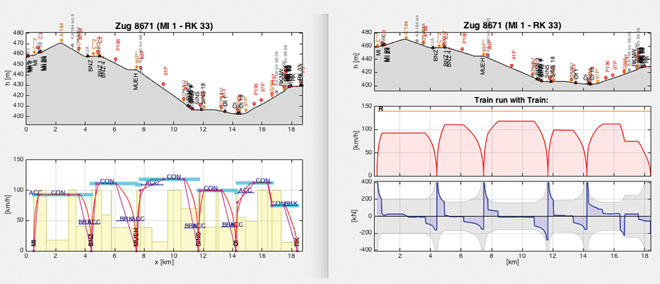
Figure 7: SBB’s head check forecast: the data laboratory calculates the speed profiles travelled from various data sets
The topological profile of a train’s movements; the positions of various safety assets; and the necessary stopping times are used to calculate a speed profile for the specific composition of passenger multiple units (see Figure 7 (left-hand side)). The traction force is calculated on the basis of the speed profiles. This means that sections of the track which are at particular risk of developing faults can be identified more effectively and reserves can be released from noncritical sections. SBB, therefore, has access to a new sensor system that not only provides descriptions of loads, but of stress too. The actual forces applied to the track by trains can be measured objectively in kN for precise locations (see Figure 7 (right-hand side)).
Summary and outlook
In developing swissTAMP, SBB has laid the foundations for a framework that enables existing and future data flows to be systematically analysed, thus permitting the efficient forward planning and execution of track maintenance. The head check forecast example demonstrates the potential that lies in existing data. swissTAMP will be rolled-out for operational use in 2017. The transition from reactive to proactive asset management will therefore also be anchored in operations.
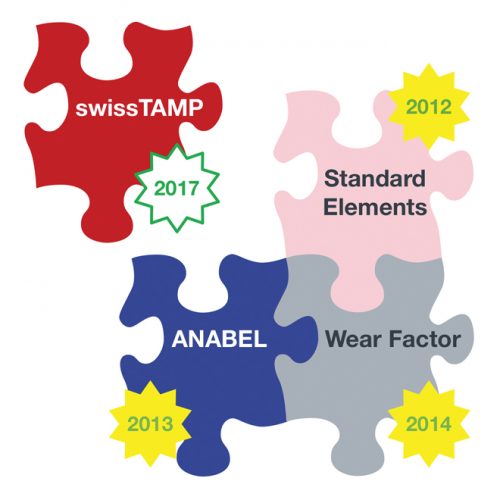
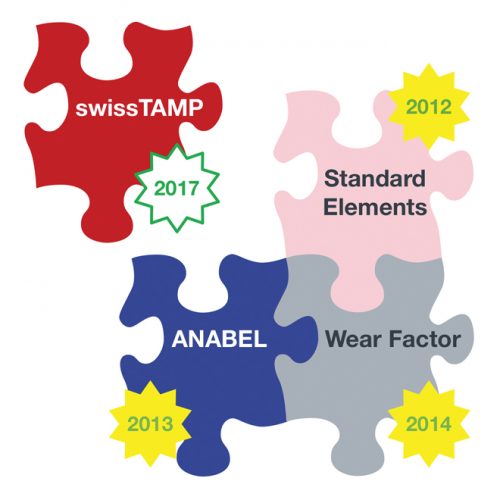
Figure 6: Systematic development of asset management tools
By forecasting asset behaviour and performing calculations on the basis of life-cycle costs, sustainable reductions in maintenance costs can be achieved. Through swissTAMP, SBB is boosting preventive maintenance to stop damage from occurring in the first place.
References
- SBB Infrastructure: Annual summary report 2015 on the LV 2013–2016, P. 21 (2016), http://www.sbb.ch/files/infrastruktur/sbb_themenlandschaft/files/
ZusammenfassenderJahresbericht2015SBBInfrastruktur_de.pdf - Holzfeind, J.: Die Herausforderungen des Anlagenmanagements Fahrbahn SBB und dessen Massnahmen zur Beherrschung des steigenden Verschleisses. WASCOSA Infoletter; Issue 20 (12/2012)
- Renggli, R; Holzfeind J.; Marschnig S..: Netzweite Investitions- und Instandhaltungsstrategie im Bereich Fahrbahnoberbau der SBB. ETR, 61 (2012) P.10-17
- Winklehner, D.: Modell für die strategische Mengenprognose vom Substanzerhalt der Fahrbahn. ZEV Rail, 139 (2015) 4th edition
- Riessberger, K.: Gleisgeometrie und Wirtschaftlichkeit – oder – wie gut muss ein Gleissein? ÖVG Spezial, Volume 41 (1998)
- Mlinaric, T.: Long-term assessment of track geometry quality with the aim of maintenance demand identification. Dissertation, University of Zagreb in cooperation with Graz University of Technology (2001)
- Auer, F.: Ein Prognosemodell zur Abschätzung der Nutzungsdauer des Oberbaus unter Berücksichtigung der Eingriffsschwelle. Dissertation, Graz University of Technology (2003)
- Holzfeind, J. and Hummitzsch, R.: Zur Prognostizierbarkeit des Qualitätsverhaltens von Gleisen. Dissertations, Graz University of Technology (2009)
- http://hadoop.apache.org (as per 26.9.2016)
- Nerlich, I.; Holzfeind J.: ANABEL – ein detailliertes Belastungs- und Beanspruchungsmonitoringsystem mit grossem Potential. ZEV Rail (11-12/2016)
- Nerlich, I.; Holzfeind J.: Wie wirtschaftlich ist gleisschonendes Rollmaterial“.. wie einen Verkehr von Morgen in der zukünftigen Auswirkung auf Gleisinstandhaltungenbeziffern?. 42nd Conference on “Modern Rolling Stock” (2014).
since 2013 in his capacity as Chief Project Manager. Heading-up the data laboratory, Krzysztof is also involved in the agile transformation of SBB.
The rest of this article is restricted - login or subscribe free to access
Why subscribe? Join our growing community of thousands of industry professionals and gain access to:
- Bi-monthly issues in print and/or digital format
- Case studies, whitepapers, webinars and industry-leading content
- Breaking news and features
- Our extensive online archive of thousands of articles and years of past issues
- And it's all free!
Click here to Subscribe today Login here